TECHNICAL REPORT
Grantee |
TeleMARS Pty Ltd.
|
Project Title | Training and Knowledge Sharing: Network Analysis for AI Transformation |
Amount Awarded | USD 85,000 |
Dates covered by this report: | 2021-12-14 to 2023-12-31 |
Report submission date | 2024-01-22 |
Project leader name |
Chen Lin
|
Project Summary
While network technologies and architecture evolve rapidly, the complexity of Network Operation management has grown significantly over the past 20 years. The Network Operation industry has experienced increasing challenges:
- Lack of collaboration and coordination across teams.
- Fast pace of change in the cloud and dynamic resource orchestration means that knowledge and information is usually not up-to-date for troubleshooting problems.
- Troubleshooting is time consuming because it often involves correlating data across multiple devices and tool sets and requires manual processes to arrive at sound diagnoses.
- The ever-growing cyber-attacks impose on-going threats on network operations.
Meanwhile, emerging Artificial Intelligence (AI) solutions have been studied, experimented and proven effective to resolve network operation problems such as detecting cyber-attacks. However, to adopt AI technologies to create benefits, the Network Operation industry needs to enhance its capabilities across various professional disciplines and community groups.
The original goals proposed by this project were targeting at the professionals in network operation or management industry in Asia-Pacific region to:
- Strengthen knowledge sharing across communities of practice such as Network Operator Groups (NOGs) and Computer Emergency Response Teams (CERTs).
- Enable easily accessible and scalable training and mentoring resources and opportunities on AI related subjects to support capacity building and professional development across the Asia Pacific region focusing on network operations and security.
- Provide scalable facilities to support on-going training, learning, knowledge update, and peer reviews on network data analysis across communities of practice.
- Improve professional capabilities on the areas of diagnosis, monitoring, measurement and/or analysis of live or historical datasets related to Internet infrastructure and its security across the Asia Pacific region.
- Contribute to the on-going improvements in Internet operation and security across the Asia Pacific region.
Table of Contents
- Background and Justification
- Project Implementation Narrative
- Project Indicators
- Project Review and Assessment
- Diversity and Inclusion
- Project Communication
- Project Sustainability
- Project Management
- Project Recommendations and Use of Findings
Background and Justification
TeleMARS completed a research project “Experiment and improve reinforcement learning algorithms to enhance anomalous network behaviour detection” which received an ISIF Asia 2020 grant. Through this project, TeleMARS team has identified that AI methodologies have strong capability in resolving cyber-attack detection problems. This finding is consistent with the latest research and industry trend of applying AI solutions in network operations and management to overcome the growing challenges. The findings and the lessons learnt also recommended that the development of effective AI solutions requires a number of prerequisites to be in place, including comprehensive and clean real network data, accurate interpretation and suitable construction of network data for a target problem.
The TeleMARS team also learned that network technologies, devices and architecture were changing rapidly in recent years, the complexity of network management and monitoring increased dramatically. There was interest in knowing how the AI technologies can be applied to resolve the problems and improve service efficiency. The TeleMARS team observed increasing needs of data and AI capability across the communities of practice who are at the front line facing the network management and security challenges. To be AI ready, the professionals in network operations and security need to equip themselves with new knowledge, skills and learning facilities.
As a consequence of global pandemic and the new trend of remote working, organizations are struggling more than ever to manage competing network resources, growing user demands, complex troubleshooting challenges, new digital transformation initiatives and technologies. IT directors are doubling their efforts to find effective ways to improve the network while reducing operational costs. The AI for IT Operations (AIOps) concepts and approaches, which combines big data and machine learning to automate IT operations processes, have emerged with promising potential to resolve the challenges.
From the previous project experience and the discussions with the experts from RMIT University, we understood the importance of knowledge sharing and skill improvements in the area of diagnosis, monitoring, measurement and analysis of live or historical real network datasets including emerging cyber-attacks. This will support the development of effective AI solutions and equip network professionals with the required knowledge.
The emergence and evolution of ChatGTP inspired more attention on the development of AI. People are questioning what AI would lead us to, what are the impacts and benefits. In the meantime, more research has been carried out to explore the methodologies that may overcome the challenges of the rapid changes in network environments. While the project progressed, TeleMARS team discovered there is a shortage of skills in network data analysis in current network operation community and there is an increasing need for learning about the basic knowledge about AI Analytics to understand what is relevant to the industry and how people could prepare for the on-going development of AI technologies.
The project team is motivated to develop innovative training approaches and facilities to enable knowledge sharing and skill improvements in network data analysis area, aiming to support AIOps transformation that benefits Internet development.
Project Implementation Narrative
Problem statement
The original problem statement drafted at the time the proposal submitted for funding was as below.
From the previous project, we identified that data driven AI solutions are highly sensitive to specific network environments. To develop and implement solutions that can effectively resolve the real-world problems, we need to analyze the specific network data and prepare the data in the correct structures for the application of algorithms.
The capability of network data analysis and processing is an essential enabler in AIOps ecosystem which supports successful implementation of AIOps solutions. The development of the network data analysis capability requires the industry to upskill and equip the workforce with new knowledge, skills and facilities.
The upskilling of current workforce faces the following challenges:
- Network data analysis requires a combination of expertise in network engineering and data science;
- The accessibility to the required expertise is limited;
- Due to the rapid evolution of network environments, the knowledge of network data changes quickly which requires mechanism of continuous knowledge update; and
- The lack of tools for non-data-science professionals to learn, collaborate and share knowledge.
Implementation
There were three major work streams of project implementation:
- Stream 1) community engagement and workshop promotion;
- Stream 2) training workshop preparation and delivery; and
- Stream 3) the development of a network analysis training platform.
The work of the three streams was carried out in parallel but progressed at different paces due to different challenges.
Stream 1
The community engagement stream included the work activities of
- Sending messages and reaching out to the community through various campaigns;
- Receiving feedback via both questionnaires and direct feedback;
- Registration of interest; and
- Converting interest to participation. The target audience were the Network Operation professionals across Asia-Pacific region.
Email list campaigns were conducted in 2022. In order to make sure the training framework and approaches developed through this project met the needs of professional practice, the project team engaged Network Operation professionals to understand their upskilling needs and their preferences in training approaches. Initial community engagement was conducted with Network Operator Groups (NOG) in the Asia Pacific region, APNIC-talk lists and ISIF Asia grantees to receive feedback and register interest.
By August 2022, 48 network operation professionals from different areas of the Asia Pacific region were engaged in the communication and provided feedback to the constructed surveys. The feedback showed:
- 98% of the responders were from network and system engineering discipline
- 35% of them had cybersecurity expertise
- 58% worked in technical support or operational maintenance, while 25% of responders also held management or commercial roles.
Regarding network data analysis experience:
- 10% of responders claimed they had no experience
- 60% considered themselves beginners
- 28% of responders had intermediate level skills
- 2% claimed they were highly experienced
- 16% of responders had not heard about AIOps
- 70% of responders knew little about it
- 14% of responders had some knowledge about AIOps
So far no one had claimed having strong knowledge of AIOps. 100% of responders expressed strong interest in gaining or improving the knowledge and skills in both network data analysis and AIOps while 30% responders might encourage their teams to upskill in these areas.
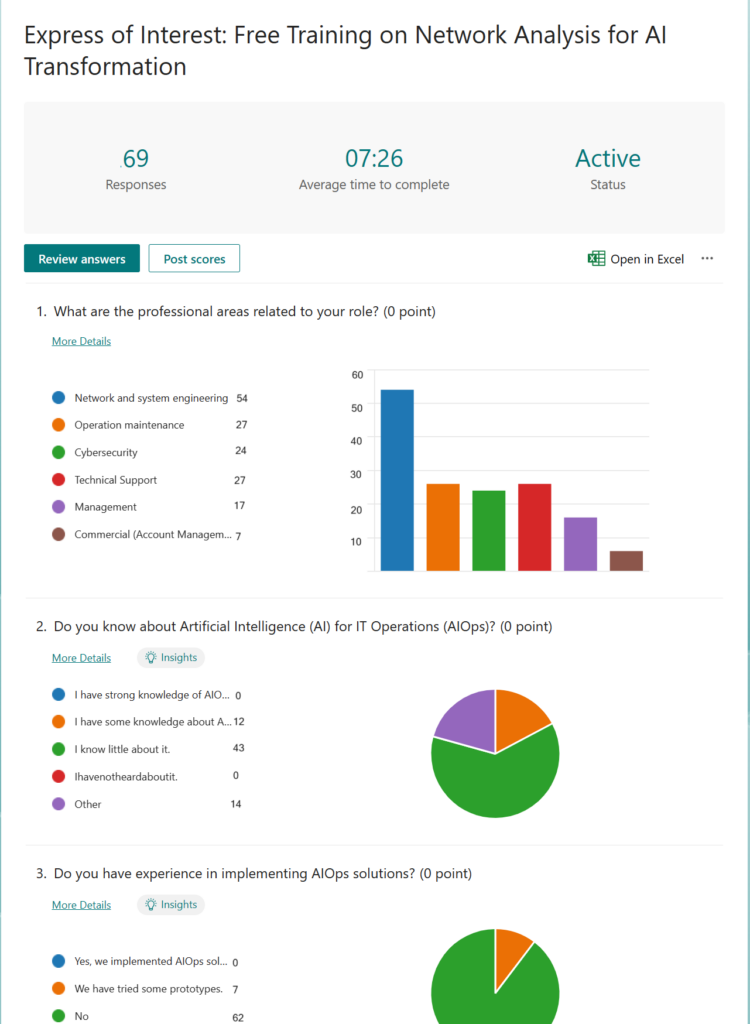
The feedback provided strong evidence which not only supported the initiatives of the project - “Training and knowledge sharing: Network analysis for AI transformation” but also identified the industry needs of learning and adopting AIOps methods and technologies.
Based on this positive feedback, a number of knowledge-sharing workshops were delivered from October 2022 to February 2023. However, the actual workshop participation rate was very low. Despite the positive feedback from community, there were only 1 or 2 participants turned up in each workshop with total of 3 people participating during the timeframe.
In order to improve the participation rate, the project team engaged a marketing specialist to conduct website campaigns and promotions on Queensland Government ICT research panel between February and June 2023, which reached over 3,000 target audience who were ICT professionals. 21 people registered interest. However, within the 21 registered responses, only 2 people turned up in a workshop.
From August to December 2023, the project team worked with another marketing consulting service that specialized in workshop campaigns. A specific workshop campaign funnel was developed aiming to increase the number of leads. Facebook campaign strategy was adopted in order to reach a wider community.
Two campaigns were carried out between September to December 2023. The campaigns reached over 120,000 audience with 120 registered leads. Total of 17 people participated the training courses.
What was achieved in Stream 1:
Overall, the project reached over 140,000 people, engaged 188 registered audience and acquired 22 workshop participants.
Though the number of participants was limited, the feedback from participants was highly positive. All participants expressed that they had learned useful knowledge through the training workshops. The most important gain for all the participants was learning how AI works in Cybersecurity.
Website campaign page: https://www.telemars.com.au/network-ai/
Supporting documents that are attached to this report include 1) aggregated feedback results, 2) feedback result screenshot, 3) survey form, 4) Facebook ad report screenshots, 5) Facebook campaign creation screenshot, 6) campaign funnel screenshot, 7) communication letter, 8) training workshop promotion post, 9) QLD ICT Research panel campaign screenshot and 10) campaign post.
Stream 2
Meanwhile, TeleMARS engaged RMIT University (RMIT) to detail the training partnership and services under the Memorandum of Understanding to collaborate on training workshop preparation and delivery. The experts from the School of Computing Technology and the Centre for Cyber Security Research and Innovation worked with TeleMARS team to work out the alignment between two parties.
From August 2022, two teams defined the scope of work including the syllabus of training courses, methods, use cases, and demonstrations. The contract finalization process took longer time than expected. The two teams kicked off the collaboration in February 2023 and concluded the work delivery in July 2023.
The two teams worked on a same workspace through Microsoft Teams sharing files to ensure everyone was working on the same version. Team members promptly discussed any issues through Teams chat and calls. Weekly meeting was carried out to make sure the consistent progress and solve any issues.
The work of Stream (2) included a) the development of presentation slides for training workshops; b) the development of use cases, datasets, Analytics and AI models; c) training workshop delivery to the participants acquired through Stream (1) activities.
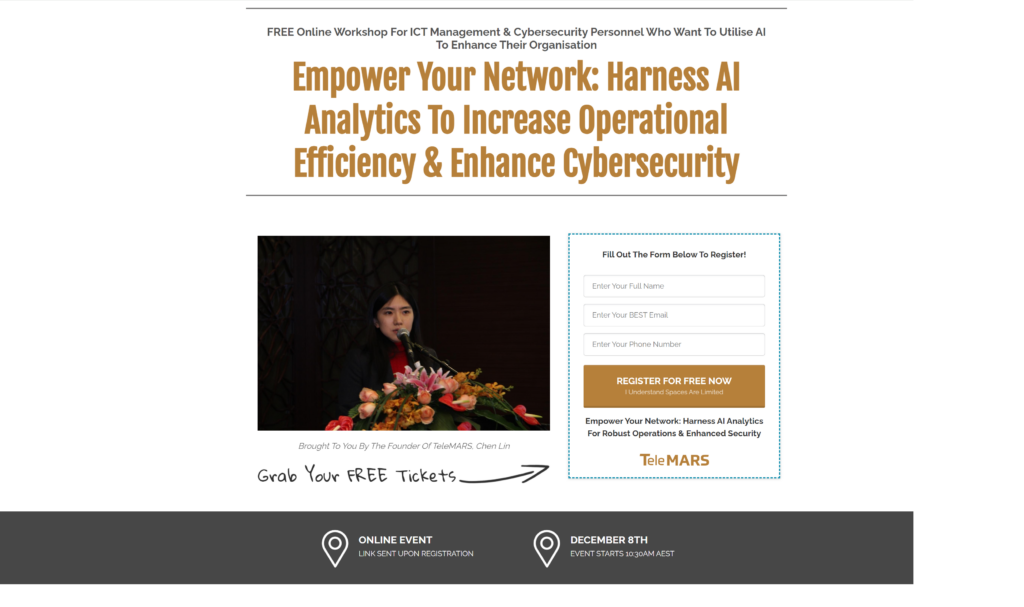
The workshop contents were designed to cater the most needed knowledge and skill practice to the trainees. The objectives included enabling the trainees to improve their network data analysis skills and capabilities, to understand process of network data analysis, to understand what are involved in adopting AIOps technologies and solutions.
a) The subjects of the courses delivered are outlined below.
- Introduction of network traffic data including the definitions, data structure.
- Introduction of real-time network data collection.
- Introduction of network data analytics.
- Introduction of AI in Cybersecurity, the concepts and relevant technologies.
b) Use Case Development.
The use cases are explained below.
1. Bandwidth utilization analysis use cases were developed to demonstrate how analytics methods can help analysis and bandwidth utilization and identify bottlenecks.
Three network traffic datasets which were generated through a simulation environment were developed.
The analysis of bandwidth by source/destination, time, protocols and payload was conducted with six analysis scripts developed. The scripts were used in training workshops.
2. A machine learning model for intrusion attack detection was developed.
Canadian Institution of Cybersecurity ("CICS") dataset was used in this use case. A machine learning model was trained and deployed to the training platform. The model was tested on the training platform with high detection accuracy. The model then was used in training workshops.
3. A machine learning model for malware attack detection was developed.
A malware dataset from CICS was adopted. A machine learning model was trained and deployed to the training platform. The model was tested on the training platform with high detection accuracy. The model then was used in training workshops.
The training materials were developed jointly by TeleMARS and RMIT teams. Use cases, sample datasets, data processing scripts, Analytics models and AI models were validated and implemented on the Network Analytics platform. The training workshops were delivered jointly.
What was achieved in Stream 2:
The materials of training courses were developed and largely met the requirements. Use cases including AI Analytics models for training activities were developed and largely met expectations.
Training workshops were delivered with live demonstration on the Network Analytics Platform. The participants did some practice on the platform during the workshop using the use cases.
Limitations: Due to the limited number of participants, peer reviews and discussions were not conducted. The quality of the workshop slides could be further improved.
Supporting documents: 1) Scope of work - "Network Data Capability Enhancement RMIT Collaboration". 2) Workshop slides.
Stream 3:
TeleMARS technical team developed a cloud-based network analysis training platform. The processes included project planning and management, designs, system configuration, team building, development environment configuration, software development, continuous testing and deployment. Iterative development approach was applied to gain quick feedback to adjust or improve the functionalities.
The development work took more time and resources than initially planned. This is a common challenge in software development. TeleMARS continuously enhanced team building to ensure the stable delivery and maintenance of the network analysis platform.
An MVP of the training platform was developed by end of 2022. Further testing and improvements were carried out during early 2023.
The MVP supported the end-to-end process of network analytics using both data analytics and AI solutions. A high-level conceptual diagram of the functional components of the platform and screenshots are attached to this report.
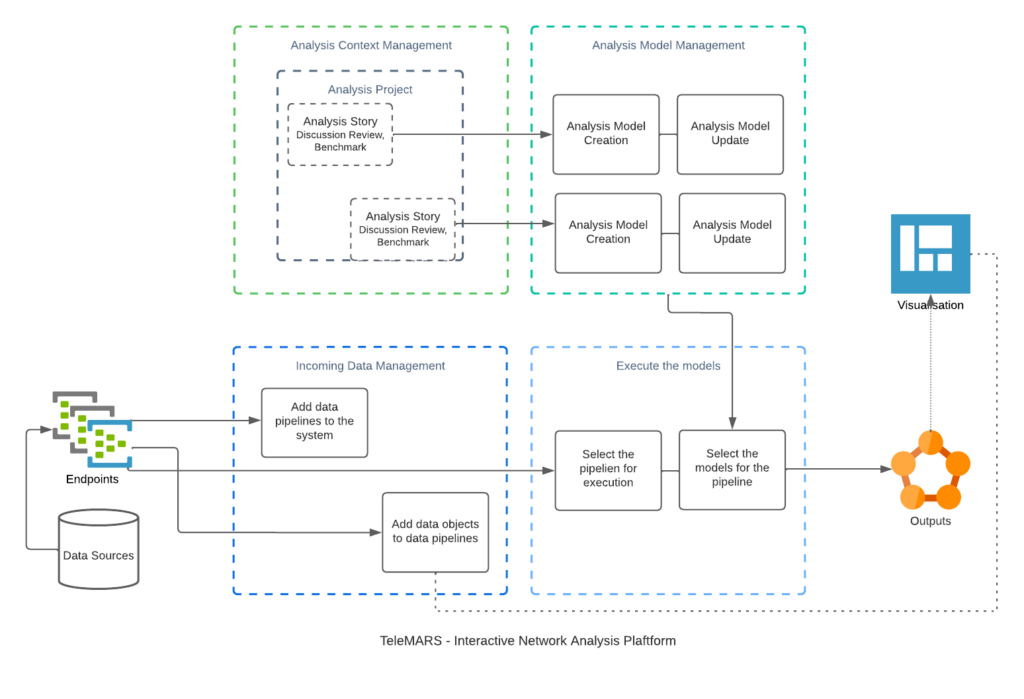
What was achieved in Stream 3:
An MVP that works in a controlled environment was developed and validated. RMIT project team implemented the use cases on the platform and delivered the expected results. The platform was used during the workshops in 2023 for live demonstration and controlled practice.
Limitations: The platform is not at the level for commercial usage. It has limited capacity for a large number of users, large volume of data, and a large number of concurrent transactions. The security measures are not at the level for open access.
Beginning of Project
Activity | Description | #Months |
---|---|---|
Community engagement | The project team conducts engagement campaigns across communities of practice in the Asia Pacific region to promote the project, gather feedback, register interest and membership of participation. The process of the campaigns involves survey, questionnaires, social media interactions, meetings, and workshops. The engagement outcomes are recorded and analysed to support the downstream activities. | 5 |
Establish an interactive Network Analysis platform | An existing cloud-based data analytics product which was implemented to execute the anomaly detection model experiment and evaluation in the previous ISIF 2020 grant project is adopted to setup a platform supporting the proposed training and learning. The platform enables live demonstration and exercises of network data analysis, as well as knowledge sharing and peer review. | 5 |
Remainder of Project
Activity | Description | #Months |
---|---|---|
Training and mentoring | Training on how to use the interactive Network Analysis platform and the tools is provided to the registered participants. Professional training workshops on network traffic analysis, incident diagnose, performance measurement, and cyber-attack analysis are delivered using the Network Analysis platform. The workshops also teach how AI technologies can enhance network operations and how the analysis outcomes support the development of AI solutions. The training and mentoring are designed for the professionals in the fields of network operations and security to support their capacity building and professional development. | 11 |
Network data analysis exercise | Selected real network datasets are made available on the Network Analysis platform. The registered participants have access to visualise data, observe benchmark analysis examples and results, practise analysis and improve skills. The analysis activities include managing data quality, analysing network traffic, diagnosing incidents, identifying anomaly behaviours, and measuring performance. This activity starts in parallel with the training and mentoring activity. | 8 |
Peer review and Knowledge sharing | The data analysis exercises and the results can be reviewed by other registered participants. Workshops or forums are organised to discuss on the following topics: • the analysis and review results and outcomes; • how these outcomes could support the development of AI solutions; and • how AIOps can improve the efficiency and the performance of network operations and security. The benchmark examples, baselined cases/scenarios, analysis and review results, and the discussions are shared with wider communities through various communication channels. This activity starts in the middle of the network data analysis exercise activity. | 8 |
Project Indicators
Indicator | Status |
---|---|
The project reached hundreds of community members and collected meaningful feedback (40-70) to support workshop design during the initial community engagement. | Completed |
An MVP of an interactive Network Analysis platform was developed with the required functionalities to support the project activities and objectives. | Completed |
1) The proposed training workshops were developed; 2) The use cases were developed and implemented on the platform. 3) The workshops were delivered and achieved learning objectives. | Completed |
1) Project teams collaborated on the Network Analysis platform. 2) Participants used the Network Analysis platform to conduct analysis successfully. 2) 80% of the trainees completed training tasks. 3) 100% of trainees make improvements in their skills. | Completed |
1) The project reached over 100,000 target audience. 2) Within the reached audience, about 200 registered interests. 3) 20 to 40 people participated in the training workshops. | Completed |
Indicator: The project reached hundreds of community members and collected meaningful feedback (40-70) to support workshop design during the initial community engagement. Status: Completed Start and End Dates: February 15, 2022 to July 31, 2022 Description: Baseline:There was no community engagement of this initiative prior to the commencement of this project. There was no accessible data demonstrating the needs of learning AI Analytics in Network Operation community. Activities: The community engagement campaigns included 1) Engagement plan 2) Communication material preparation 3) Communication channel building 4) Conduct communication and engagement through the channels 5) Collect feedback 6) Analyse feedback to inform implementation decisions 7) Registration and enrolment. Outcomes: The email list campaigns reached hundreds of people in the community. 48 members at different positions from different areas of Asia Pacific region provided feedback through an online survey. The feedback showed strong interest in skill improvement in the subjects of Network Data Analysis and AIOps. The project received registration of interest from the 48 community members with intention to participate in the project activities during the initial engagement. |
Indicator: An MVP of an interactive Network Analysis platform was developed with the required functionalities to support the project activities and objectives. Status: Completed Start and End Dates: February 1, 2022 to January 31, 2023 Description: Baseline:A data analytics pipeline with the collaborative data processing and analysis functionalities was built during the development and experiment of the anomaly detection models in the previous project. Activities: The key activities included solution architecture design, environment configuration, software development, testing, deployment and release. The work included the following key components: 1) enhancing the technical architecture to satisfy the needs of live demonstration of AI models using large dataset. 2) new features that satisfy the specific requirements of the Network Analysis training needs. 3) enhancement on existing functionalities to support the development of a wider range of analysis and AI models. Outcomes: The key functionalities of the network analysis platform supported the training workshop delivery. The designs were proven to be effective in supporting the learning of AI Analytics. The platform supported the collaboration of a diverse project team which involved subject matter experts, data engineers and scientists. It was used in work Stream 2) use case development and enabled the end-to-end use case development, testing, and implementation. The results were as expected. The platform was then used in workshop to deliver live demonstration and support training activities. The functions worked well for the purposes of this project. |
Indicator: 1) The proposed training workshops were developed; 2) The use cases were developed and implemented on the platform. 3) The workshops were delivered and achieved learning objectives. Status: Completed Start and End Dates: October 1, 2022 to December 8, 2023 Description: Baseline:There was no accessible data showing similar workshops delivered to Network Operation community prior to the commencement of this project. The feedback from the initial community engagement showed there were demands of learning basic knowledge of network data analytics and how AI works in cybersecurity. Activities: 1) Workshop planning. 2) Workshop material design and preparation. 3) Workshop environment preparation. 4) Workshop delivery. 5) Q&A, feedback and discussions. Outcomes: Participants were highly satisfied with the workshop contents and delivery method. They appreciated that they have learnt substantial knowledge about how AI Analytics works to improve network performance and security measures. The training course contents were proven effective for the majority if community members to start the journey of equipping themselves with the right knowledge. There is still room to improve the quality of the contents and the contents will need to be continuously adjusted to catch the rapid development of AI Analytics technologies. |
Indicator: 1) Project teams collaborated on the Network Analysis platform. 2) Participants used the Network Analysis platform to conduct analysis successfully. 2) 80% of the trainees completed training tasks. 3) 100% of trainees make improvements in their skills. Status: Completed Start and End Dates: April 1, 2023 to December 8, 2023 Description: Baseline:Such analysis exercises was not available prior to the commencement of this project due to lack of such platform. Activities: 1) The trainees were given instruction of how to use the platform effectively. 2) Real network datasets were prepared for the exercises. 3) Use cases including benchmarks, and assignments were created. 4) Trainees conducted analysis exercises progressively from executing basic analysis models to running AI models. 5) The analysis results were evaluated by instructors during the workshops. Outcomes: The platform supported the collaboration of a diverse project team which involved subject matter experts, data engineers and scientists. It was used in work Stream 2) use case development and enabled the end-to-end use case development, testing, and implementation. The results were as expected. The participants practiced the implementation of the use cases using the platform in a controlled environment. Such hands-on practice enhanced the learning experience and outcomes. |
Indicator: 1) The project reached over 100,000 target audience. 2) Within the reached audience, about 200 registered interests. 3) 20 to 40 people participated in the training workshops. Status: Completed Start and End Dates: October 1, 2022 to December 8, 2023 Description: 1) the number of target audience reached; 2) the number of registered interest and feedback provided; 3) the number of participants who participated the training project and achieved learning objectives. Baseline:The initial communication reached hundreds of target audience using email list method. 48 people registered interest and provided feedback. Activities: Multiple marketing campaigns were developed and carried out from October 2022 to December 2023. Communication and engagement were managed throughout the process. Outcomes: The project reached a wide range of target audience. Over 140,000 people were reached. 188 registered interests were achieved. 25 participants participated in training workshops. |
Project Review and Assessment
Summary:
This project proved the concepts of the original goals.
It largely achieved the expected number of reaches and engagements in Network Operation community.
It identified the needs of knowledge-sharing and learning in the space of network data analytics and AI.
The method of training and learning materials were effective in improving the capabilities of using AI Analytics to monitor network performance, diagnose issues and improve security measures.
The network analysis training platform provided facilities to support the training and learning. It could be further improved and used in a wider range of learning activities.
This project contributed to Internet development by achieving the following outcomes.
1. Delivered the messages about using AI Analytics to make improvements in Network Operation and raised awareness in over 140,000 target audience in the community.
2. Developed and delivered effective training workshops the participants.
3. 22 participants learnt basic knowledge in AI Analytics in Network Operations and improved their skills. They would be able to make improvements in their work, improve Internet performance and security, and introduce the knowledge to people around them.
4. The project experimented a new concept and method of knowledge sharing using use cases and cross-discipline collaboration. It developed and proved an effective approach and framework that enable the learning and upskilling in AI Analytics in Network Operation community. The method and framework could be used for skill enhancement for Internet performance and security.
5. The project team developed approaches and pathway to make industry-research collaboration possible. The experience learnt could continuously support future technical capacity enhancement for Internet development.
Findings and Outcomes
1. This project found there is no shortage of interest in AI Analytics in Network Operation community. With the increasing awareness of AI technology development, the demands of using AI Analytics to improve network performance and solving cybersecurity challenges are growing quickly.
2. The current needs of knowledge sharing and learning include the knowledge about network data, intermediate knowledge about analytics methods, and the basic concepts about AI and how it works. There was a great interest in understanding how AI may impact the industry in a short term and longer term.
3. The training course contents were proven effective for the majority of community members to start the journey of equipping themselves with the right knowledge. There is still room to improve the quality of the contents which will need to be continuously updated to catch the rapid development of AI Analytics technologies.
4. The key functionalities of the network analysis platform supported the training workshop delivery. The designs were proven to be effective in supporting the learning of AI Analytics. The platform supported the collaboration of a diverse project team which involved subject matter experts, data engineers and scientists. It was used in work Stream 2) use case development and enabled the end-to-end use case definition, testing, and implementation. The results were as expected. The platform was then used in workshop to deliver live demonstration and support training activities. The functions worked well for the purposes of this project.
The participants were able to practice the implementation of the use cases using the platform in a controlled environment. Such hands-on practice enhanced the learning experience and outcome.
5. Collaboration was proven an effective method in building AI Analytics capabilities in Network Operation industry. The collaboration between research institution and industry enabled the transfer of research outcomes and expertise as well as the sharing of industry experience. This was important format to ensure the development of training courses integrated industry needs with the research experience and latest studies. Such collaboration ensured the effectiveness and relevance of the training contents and materials and the satisfaction of participants.
To use and promote the outcomes of this project, we plan the following potential activities: a) sharing the project outcomes with a wider community at conferences or seminars; b) sharing learning materials with a wider community that is easy-accessible; c) working with organisations to make the learning process in the scope of the roles.
Lessons Learned
1. Despite all the campaign effort and the good number of outreach and interest, the biggest challenge this project encountered was the low participation rate. The feedback showed most people struggled to stick to the time commitment to free training workshops while they were distracted by other life and work commitments. It was easy for people to show their interest, but it was also easy for people to be distracted. For people who have a full-time job, it was difficult for them to keep the commitment to a free training workshop while their work commitments were always at a higher priority.
Moving forward, we need to develop an effective and practical approach to enable people who have interest to participate without being burdened or distracted by other commitments.
The potential methods may include self-learning online courses which could be taken anytime that is suitable for the participants. However, this method may not work for the people who prefer interactions and guidance. Otherwise, the upskilling could be integrated into business-as-usual work arrangements.
2. The capacity of the network analysis platform was not sufficient to cope with large number of users or large volume of data. The volume of data or the transactions of analysis could increase quickly which needed to be carefully considered in the technical designs.
3. We learnt that it was not easy for users who did not have strong data analysis and/or AI experience to use the platform without close guidance. The platform user interface needs to be more user friendly. Detailed instructions were needed.
4. The establishment of industry-research collaboration took a lot more effort and time than expected despite the strong willingness and mutual understandings from both parties. We learnt that it was not easy for research institutions to make quick changes in their existing agenda and framework. It is likely there are gaps between the objectives of a collaboration project and those of research. In order to build an effective collaboration, both sides need to invest sufficient time to align the objectives and agendas across multiple levels from project teams to management and executives of the institution. The project teams should also allow sufficient preparation time to make sure the alignment of project methodologies, management and the availabilities of team members.
Local Technical Capacity and TeleMARS Capacity Improvements
- Technical Team. Local talents were engaged to develop network analysis platform which improved the technical capacity of TeleMARS team.
- Skill Enhancement. The use case development and implementation processes enabled the research team to learn industry practice and improve their skills of applying research experience in industry applications. Same process enabled TeleMARS project team to learn about research methods and outcomes and enhance skills in AI.
- Research-industry Collaboration. The collaboration framework not only enabled the project teams to improve their knowledge and skills, but also enables on-going capacity improvements for both RMIT and TeleMARS teams.
- Capacity in Community Engagement. TeleMARS team learnt various methods and techniques of engaging community members and promoting events. These are important skills we need to further promote the outcomes of this project and create larger impacts on Internet development.
The Potential for Growth
- Learning Infrastructure. The outputs of this project including the workshop materials and network analysis platform established a foundation of a learning infrastructure for on-going skill enhancement. It provided a prototype of scalable facilities to support on-going training, learning, knowledge update, and peer reviews on network data analysis across communities of practice.
- Proof of Concept. This project proved the concept of the original goals to improve professional capabilities on the areas of diagnosis, monitoring, measurement and/or analysis of live or historical datasets related to Internet infrastructure and its security across the Asia Pacific region.
- Network Analysis Platform. The functionalities built during this project provided a minimum viable application that works in a controlled environment. With further improvements on the capacity and security measures of the platform, it will enable easily accessible and scalable training and mentoring resources and opportunities on AI related subjects to support capacity building and professional development across the Asia Pacific region focusing on network operations and security.
Diversity and Inclusion
As the project leader, Chen has been continuously practicing and developing her leadership skills throughout the project journey. This experience demonstrates women’s capability and potential in playing leadership roles in IT innovation.
This project has encouraged the participation of women engineers. Despite the small percentage of female engineers available in current workforce, we engaged a highly experienced female software engineer, Helen Ding, to join the project team. We supported Helen to step up taking mentoring and management responsibilities. This provided opportunity for Helen to advance her personal development and explore leadership potential.
The project team comprises professionals from diverse culture and language backgrounds across various areas of Asia and Europe. 66% of the team members speak English as a second language. Our collaboration framework encourages team members to understand and respect the differences, to learn from each other, and to learn the advantages that each culture offers.
Being open-minded, the team embraces the diversity and takes the opportunity to integrate various perspectives. The diversity inspires the team to think out-of-the-box and turns the differences into creative power.
Facebook campaigns were designed to reach diverse demographic audience by promoting female leadership. The statistics showed that about 23% of the audience reached were female, and 23.3% of registered interests were from female audience. But in the workshops, only less than 1% of participants were female.
Despite all the efforts of reaching and encouraging women to attend the workshops, the percentage of female participation was low.
Project Communication
The project implemented staged communication strategy and method.
Initial community engagement stage:
The objective was to reach a good number of community members, introduce the project opportunity, and gather feedback to understand the needs. Email list campaigns and surveys were conducted to promote the project and engage with community members. 48 responses and interest were registered to inform the decisions of the project.
The knowledge learned from the community feedback was shared with RMIT team to support their understanding in the training objectives and scope.
Training workshop stage:
The objective was to reach a wide range of audience to promote the project activities in particular the training workshops, and recruit 20 to 40 community members to participate in the workshops. Various campaigns were conducted including website promotion, Queensland government ICT research panel channels, campaign funnels, and Facebook Ad to promote the training workshops, register the interest and engage the participation. Over 140,000 people were reached, 188 of those registered interest and 25 participants attended training workshops.
The above communication activities were conducted by TeleMARS team and external professional services, partially funded by ISIF grant and TeleMARS cash contributions. The outcomes of the activities were measured by the number of registered interest and the number of actual training workshop participants.
Communication strategy and plan of sharing project outcomes and outputs:
The objective is to reach a broader community to share the outcomes and lessons learnt from the project and engage more people to practice on the Network Analysis platform.
TeleMARS will submit a paper to APRICOT 2024 to share the project outcomes and lessons learnt.
TeleMARS will continue enhancing campaign funnels to reach a broader audience to share the project outcomes. Meanwhile the audience who registered interest in the previous campaigns will be retargeted for further engagement of using the Network Analysis platform.
Knowledge sharing workshops will be hosted on Humanitix platform to share the knowledge and lessons learnt from the project and engage the participants to use the Network Analysis platform.
Project Sustainability
Organization development
TeleMARS enhanced its team capabilities through the project activities including cloud platform development, AI, data analytics, multidisciplinary collaboration, marketing, engagement and project management. We improved team structure with a wider diversity and promoted female leadership at multiple levels. The team learnt and improved how to support each other to overcome challenges and achieve same goals.
The experience and skills of marketing campaign will be used to enhance the effectiveness of sharing the project outcomes with a wider community and strengthening credibility. The continuous campaigns of the project outcomes and the Network Analysis platform will be conducted which will be funded by the remaining grant and TeleMARS income.
Collaboration
The joint delivery of training workshops with RMIT team strengthened the collaboration between the two parties. The partnership was enhanced to enable future innovation activities. This project also provided a strong proof of concept of industry-research collaboration which supported the development of value-driven research and the collaborative ecosystem.
Project outcomes and outputs
The Network Analysis platform could be further developed and enhanced to support open access and be used in on-going skill enhancement for a wider community. The further development of the Network Analysis platform will be funded by TeleMARS.
The training materials including workshop slides, use cases, datasets, analytics and AI model scripts could be shared with technical community. These materials could be further developed and improved to support further advanced training activities for a broader community.
The outputs of this project can be reused for other training in the relevant topics of network management. With further updates, the materials and Network Analysis platform could stay relevant with the new industry and technology trends.
Project Management
Project administration
Project plan. Each work stream had a plan at the beginning of the project outlining tasks, timelines, resources, and the expected outcomes.
Budgeting. The project budget was managed across all the work streams to ensure the overall budget was not exceeded.
Project communication. Microsoft Teams was used as a major tool for project communication which enabled prompt discussions among team members, sharing the information and managing the consistency of versions. It is also used for project meetings.
Execution. The tasks of each work streams were implemented separately by different team members. The challenges of one stream did not impede the progress of other streams. The open discussions were encouraged to allow all team members to make contributions to the decision making at task levels. Consistent brainstorming sessions were held throughout the project delivery to integrate various perspectives and creative thinking.
Monitoring and controlling
- Performance Monitoring: The progress was monitored for each work stream and compared with the project plan respectively.
- Issue Management: Issue monitoring was conducted in each stream which identified issues as they arose to seek for the best solution and minimise negative impacts.
- Change Management: As the project encountered a number of unexpected challenges which were beyond the project team experience. Change management was applied in all three streams covering the changes in software development, professional service engagement, engagement and promotions activities, and the workshop deliveries.
- Mentoring: Mentoring and learning supports were provided to team members. This enabled the ongoing development of team capability at both individual and team levels. The growth of individual skills and teamwork capacity supported the team to better understand the problems and challenges, better utilise the strengths of each team member, and collaboratively deliver high quality solutions while keeping risks in control.
Staffing and procurement
As the project required a level of flexibility in budgeting to handle unexpected challenges, contractors were engaged to conduct platform development work. Sourcing process was straight forward as TeleMARS had existing relations with a number of contractors to satisfy the required availability.
TeleMARS team did not have the expertise in marketing and promotion, professional services were engaged to conduct activities in Work Stream 1 - community engagement and workshop promotion. There were challenges in matching the availability of the suppliers with the project timelines.
The procurement process included referral from trusted businesses and interviews to identify the service suitability including skillset, scope of services, mutual understanding of project goals, and resource availability.
Collaboration
The collaboration process was outside of business-as-usual procedures. RMIT and TeleMARS teams aligned the resource availabilities ahead of kicking off the collaboration work to avoid the disruptions caused by team member availability.
The two teams worked on a same workspace through Microsoft Teams sharing files to ensure everyone was working on the same version. Team members promptly discussed any issues through Teams chat and calls. Weekly meeting was carried out to make sure the consistent progress and solve any issues promptly.
Mentoring provided by RMIT experts: RMIT experts provided advises and mentoring in the subject of Machine Learning to TeleMARS team. This strengthened the collaboration between the two parties which enabled on-going research innovation activities.
Project Recommendations and Use of Findings
Findings and Lessons Learned
- There are increasing needs of learning how AIOps works for network operation improvements.
- The average skill level of data analytics and AI in network operation community was at beginner level. The training contents were suitable.
- Interactive live demonstration was an effective approach.
- The attendance rate of free workshop was low which was 13.3% of the registered interest.
- The industry-research collaboration enabled skill enhancement in both parties. TeleMARS team learnt and improved skills in data analytics and AI and learnt the latest research trends and outcomes. RMIT team enhanced their industry experience and the knowledge of network operation practice.
- The collaboration required alignment between two parties at multiple levels including delivery teams, project management, executive frameworks and strategies.
Recommendations
1. Industry-research collaboration
The parties should invest time and effort in aligning the objectives, guidelines, and purposes when setting up collaboration.
It is expected that the processes in research institutions may be different from industry entities. The process of project contracts and resource assignment timeframes should be considered in project planning and sufficient preparation time should be allocated prior to project commitment. Such leading time could be from 3 months to 8 months for the first time.
TeleMARS and RMIT University jointly conducted project planning which defined the scope of work including detailed tasks, expected outcomes, deliverables, roles and responsibilities. A project framework was established to support the university guidelines and objectives of both parties and manage the work progress on daily basis. A team workspace was built for the team members to collaborate, share and discuss in timely manner. Mentoring program and opportunities were provided to both teams to enable the skill enhancement.
2. Community engagement
Similar projects should plan considerable amount of time and resources to reach a wide range of target audience and attract interests.
There are a lot of distractions in this fast-paced environment. A dedicated end-to-end process from generating awareness, creating leads, to converting interest to commitment is necessary.
Professional services may be needed if project team is not experienced in promotion campaigns.
3. Learning commitment and outcomes
It is important to provide practical mechanisms to make it easy for people to take action and commit to learning activities. Such mechanisms include online training courses which are accessible anytime anywhere or incorporating the learning activities as part of organization training and the development goals.
It is worth testing various prices for training workshops as the free workshops did not secure commitment well.
As the dynamic of network operation is changing quickly, the similar projects need to pay attention to make sure the contents in particular use cases are relevant to current trends of network environment and user behaviours.
AI Analytics technologies are changing rapidly, it is important to update the use cases promptly to ensure the training is relevant to the latest technologies.
This work is licensed under a Creative Commons Attribution-ShareAlike 4.0 International License