TECHNICAL REPORT
Grantee |
Sweet Lime E-Commerce Private Limited
|
Project Title | DASH: AI and IoT Powered Data Driven Wildfire Detection and Prediction System |
Amount Awarded | USD 30,000 |
Dates covered by this report: | 2023-10-01 to 2024-03-31 |
Report submission date | 2024-05-25 |
Economies where project was implemented | India |
Project leader name |
Sakshi Ojha
|
Project Summary
Wildfires or forest fires are a growing threat with devastating consequences. This global challenge prompted the G20 nations, through the Delhi Declaration 2023, to unanimously commit to addressing wildfire prevention and mitigation efforts.
In India, about 36% of forest cover is prone to wildfires due to inadequate infrastructure, limited community involvement and human activities. We have designed and developed DASH, an AI/ML and IoT-powered unified system to detect, predict and mitigate wildfires. Our goal is to accurately predict, swiftly detect and efficiently mitigate wildfires in real-time, safeguarding biodiversity, reducing carbon emissions and fostering a sustainable future.
We have piloted DASH in Telangana’s Nallamala Forest area, which faces frequent wildfires. The pilot provided an opportunity for real-world testing and improvement of the system, working closely with the Forest Department and the community.
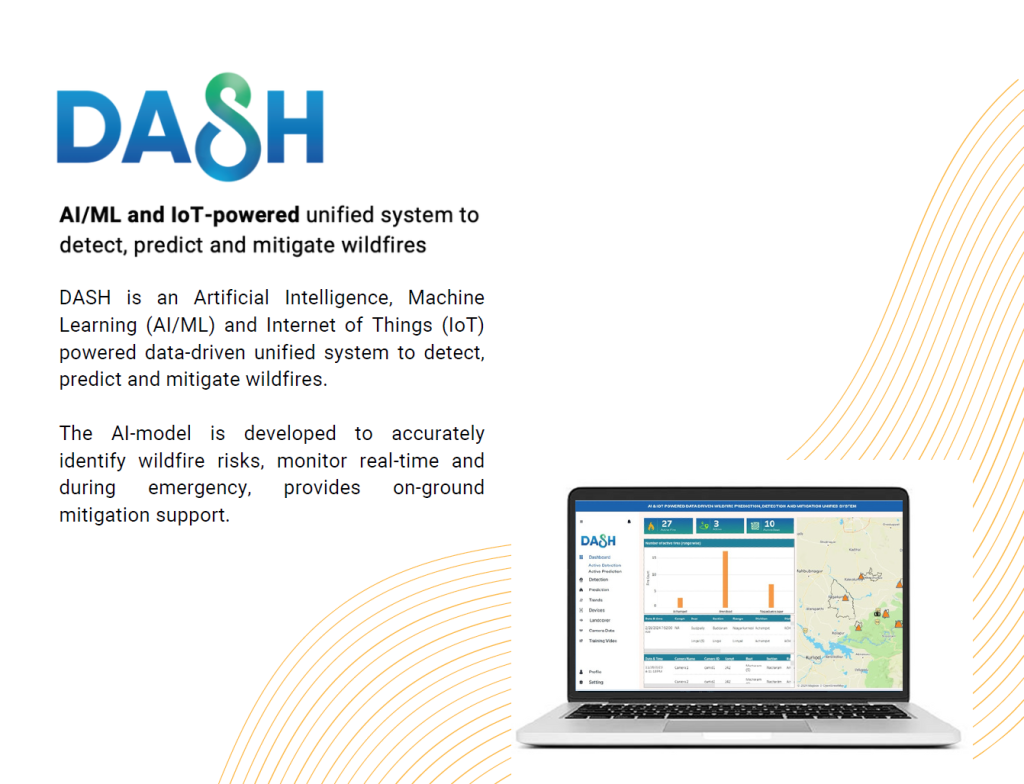
Key activities undertaken include setting up of a technical committee, conducting research, deployment of pilot testing platform, mobile application for reporting, hardware installation (LoRaWAN MESH, cameras, sensors, solar panels etc.), continuous ongoing AI/ML-model training using a diverse range of datasets (satellite, weather, landcover, wind speed, images etc.), day-to-day risk and operational management, conducting simulations, officer training and community awareness sessions. As a mitigation measure, different kinds of drones were tested to map inaccessible areas, aiding beat officers in assessing the fire through aerial surveillance and dropping water on the burnt areas.
The DASH unified system has demonstrated significant capabilities in reducing wildfire prediction and detection alert and notification times. Initially, the forest department received wildfire detection notifications within a window of 6 to 12 hours. However, with the implementation of the DASH unified system, wildfire prediction has been enhanced by leveraging a broader range of datasets, resulting in higher accuracy and reduced false alarms, both positive and negative. For instance, the integration of historical data from satellites, along with data on climate, land cover, and weather, has increased the robustness of predicting trends and achieved a 50% increase in prediction accuracy while reducing false positives in detection by 30%. Furthermore, the deployment of an on-ground detection ecosystem utilizing IoT devices, sensors, drones, and social media reporting has enabled the detection of wildfires and the dispatch of notifications with geo-coordinates within minutes, reducing detection time to less than 2 hours. Moreover, testing has shown that technology can facilitate mitigation and post-disaster management efforts effectively. Current models do not utilize sensors or other datasets but rely solely on satellite data, highlighting the advanced capabilities of the DASH system.
Following are some of our key learnings:
1. Safeguarding the forests and biodiversity is a matter of national significance, essential for the protection and preservation of both tangible and intangible assets.
2. Predominantly, wildfires at our pilot site are ground-level fires, often initiated by human activity and exacerbated by environmental conditions within the forest. As part of our efforts, we conducted testing using a variety of available data and researched to explore the potential integration of human behaviour into our AI/ML model.
3. Local communities and tourists seem to have an awareness of wildfires but exhibit cognitive dissonance regarding their habits and practices. It is important to have continuous awareness programmes.
4. Stakeholders are keen to pilot the DASH system in other forests, acknowledging the need for tailored solutions due to the unique challenges of each location. Wildfires are a priority and stakeholders are seeking an extension of the technology solution to address a range of pressing issues in forest management.

Table of Contents
- Background and Justification
- Project Implementation Narrative
- Project Activities, Deliverables and Indicators
- Project Review and Assessment
- Diversity and Inclusion
- Project Communication
- Project Sustainability
- Project Management
- Project Recommendations and Use of Findings
- Bibliography
Background and Justification
Problem:
Wildfire or forest fire is defined as “an unusual or extraordinary free-burning vegetation fire which may be started maliciously, accidently or through natural means, that negatively influences social, economic or environmental values”. A wildfire or forest fire results from a complex interaction of meteorological, biological, physical, social and human behaviour factors that influence the likelihood of a wildfire breaking out, its propagation and intensity, duration and extent, and its potential to cause damage to economies, the environment and society. These wildfires pose a growing threat worldwide, with devastating consequences felt most acutely in the Asia-Pacific region. This area is particularly vulnerable due to inadequate firefighting infrastructure, limited community involvement and a combination of human activities and geographic vulnerability. To summarize, the core problem we aim to solve is to address current challenges in predicting, detecting and mitigating wildfires, thereby contributing to reduction of greenhouse gas emissions and preventing loss of biodiversity.
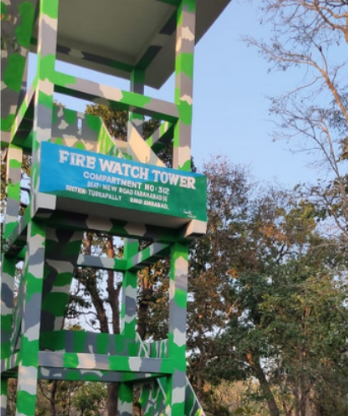
Previous attempts and approaches globally and on the pilot site:
Wildfire is a global challenge and there are multiple measures undertaken to address these. As part of our product development and enhancement, we have studied a range of available solutions in the global marketplace. The standalone products are providing solutions for either detection, prediction or mitigation. There is a clear gap in the supply of similar platforms that provide a unified approach to predict, detect and mitigate wildfires. Further, some of the solutions are cost intensive, have a technology platform lock-in and offer limited flexibility for customization.
As part of this grant, we piloted DASH in Nallamala Forest area in the State of Telangana, India. Nallamala Forest is home to the Amrabad Tiger Reserve and has diverse mammal, avian and reptilian species. In 2010, Telangana had 497 kha of natural forest, extending over 4.4% of its land area. In 2023, it lost 646 ha of natural forest, equivalent to 516 kt of CO₂ emissions. Fires were responsible for 3.2% of tree cover loss in Telangana between 2001 and 2023. (Global Forest Watch, 2024). Unfortunately, this area is highly susceptible to wildfires. Several factors contribute to wildfires in the area, including dry climate, rising temperatures, invasive weed species like Lantana camara sp., human encroachment and activities such as slash-and-burn agriculture.
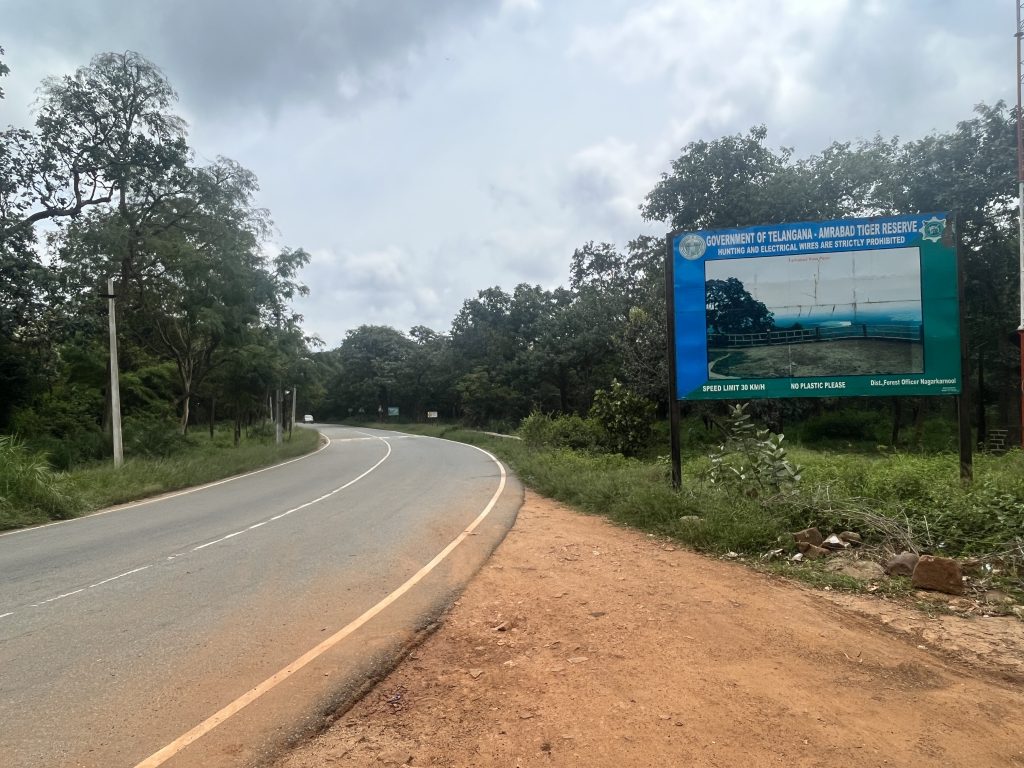
The existing methods for wildfire detection and prevention include physical watchtower guarding and satellite fire alerts from the Forest Survey of India (FSI) and National Remote Sensing Centre (NRSC). However, one of the key limitations of the current system is the long duration of detection time. The current transmission of fire alerts to the beat officer can take anywhere between 6 to 12 hours. As part of the pilot of DASH and overall enhancement of the system, our aim was to reduce the time gap to as minimal as possible by implementing solutions at the forest level and training the AI/ML model continually using appropriate data for all stakeholders in the value chain, including community members.
Further, the current FSI system does not differentiate between wildfires and other types of fires, such as waste and crop burning, leading to delays in ground validation and misinformation. Due to limited human capacity, first responders are overwhelmed by the high number of fire alerts from standalone systems, thus making timely responses challenging. Poor response leads to uncontrollable fires that pose loss to lives and habitat including prevalence of respiratory diseases due to toxic fumes, loss of homes, business, communities affected by fire and fast-tracking extinctions.
Day-to-day operations helped us with new learnings and in making changes to the project as compared to the original plan to ensure the system’s effectiveness and relevance. These changes included efforts towards input of additional datasets for improving the AI/ML model over time for better prediction and detection; enhancing the integration of forest and agricultural land cover data, beat and compartment mapping, historical records and researching how human behaviour data could be integrated into the AI/ML model.
These adjustments were necessary to adapt to the dynamic nature of wildfire risks and to maximise the impact of the project. For example, as part of our knowledge exchange session with the Indian Meteorological Department (IMD), we received valuable feedback suggesting the incorporation of higher spatial resolution maps to enhance accuracy and granularity. This includes investigating integration possibilities of open data from the Meteorological and Oceanographic Satellite Data Archival Centre (MOSDAC) of the Indian Space Research Organisation (ISRO), in addition to the NASA data being currently used in the system. IMD also recommended utilizing supplementary data such as wind direction, lightning, thunderstorm and temperature to predict the trajectory of wildfire spread. These datasets could potentially be accessed through a formal Memorandum of Understanding (MoU) as we continue to enhance our AI/ML model in future iterations.
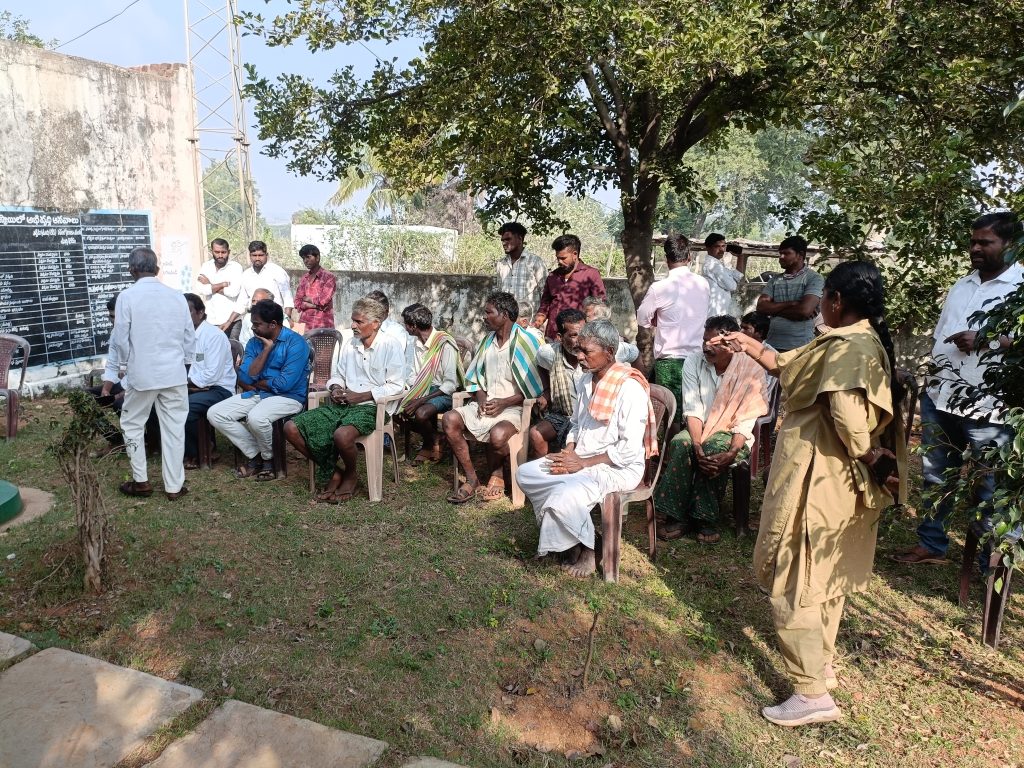
Motivation to do the project:
Our motivation to do this project stems from our recognition, awareness and commitment to develop innovative solutions for wildfire management, including for countries across Asia and Africa where the need to have affordable solutions for mitigating the devastating consequences of wildfires is more profound. With our overall organizational experience of working with UN agencies and development partners, we are cognizant of the importance and benefits of using technology for good for the environment and other sectors.
Recognizing that wildfires are becoming more widespread and a crisis spreading beyond our control, we are committed to developing innovative solutions to enhance wildfire detection, prediction and mitigation efforts. Through the implementation of DASH, an AI/ML and IoT-powered wildfire prediction, detection and mitigation system, we aim to overcome the limitations of existing methods and contributing to a more sustainable future for the planet.
Additionally, our team aims to address the shortcomings of existing wildfire management solutions with our innovative approach.
Project Implementation Narrative
Project Implementation Narrative
Response:
Our project aimed to transform wildfire prediction, detection and mitigation measures through the development and implementation of DASH, an AI/ML and IoT-powered unified system. The primary objective was to continuously work towards enhancing wildfire detection, prediction and mitigation efforts, ultimately safeguarding biodiversity, reducing carbon emissions and protecting communities from the adverse effects of wildfires. The target audience included forest departments, local tribal communities, visiting tourists and environmental organizations concerned with wildfire management and forest conservation efforts.
DASH unified system incorporates state-of-the-art AI/ML algorithms and IoT to gather real-time data on environmental conditions to predict and detect potential wildfire threats. We opted for a cloud-based infrastructure to ensure scalability and flexibility in data processing and analysis. Due to connectivity challenges in the forest, we used MESH technology to connect between devices and connect to cloud data processing platform. IoT devices were used to capture various data such as PPM, soil moisture content, temperature, carbon emission. The system was also supported with mobile application for reporting wildfires. The pilot implementation approach included ongoing rigorous testing and validation to ensure the accuracy and reliability of the system to aid the forest department for efficient action in times of crises.
As part of the grant activities, we conducted the pilot of the DASH unified system at Nallamala Forest area in Telangana. The Nallamala Forests are the largest stretch of undisturbed forest in South India apart from the Western Ghats. It is located south of the Krishna River, in particularly the section of the Eastern Ghats which form eastern boundary of Rayalaseema region of state of Andhra Pradesh and Nagarkurnool district, districts of the state of Telangana in India. The hills run in a nearly north-south alignment, parallel to the Coromandel Coast for close to 430 km between the rivers Krishna and Pennar. The Nallamala Forests is also home to the Amrabad Tiger Reserve.
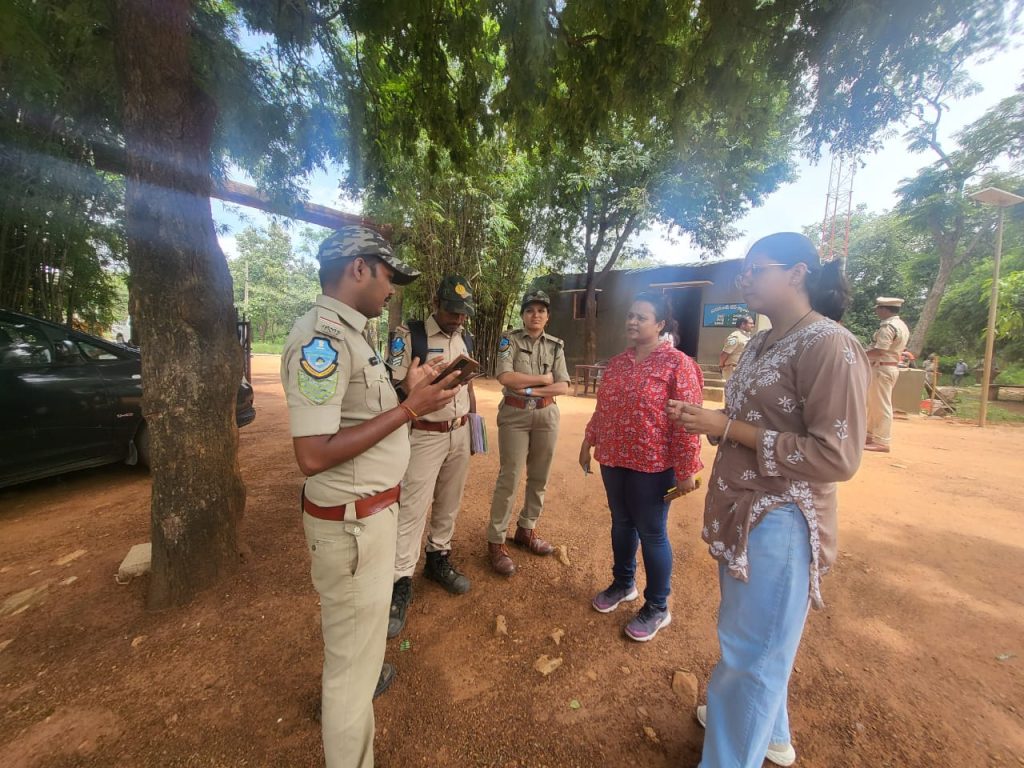
Fires have become a regular occurrence in these dry deciduous forests, from November to mid-June every year, with a peak in the months of March and April. In April 2023, approximately 11,500 forest fire alerts were recorded in Telangana. Within this expanse lies the Amrabad Tiger Reserve (ATR), extending over 2611.4 sq km, ranking India’s second-largest Tiger Reserve by core area and the sixth-largest among the 51 Tiger Reserves in total area. The Amrabad Tiger Reserve faces a high risk of forest fires, with an average frequency of ≥4 incidents per grid each year. The following are the key factors for the triggers of wildfires in the area:
● The dry and arid climate in the Deccan Plateau region (southern tropical mixed dry deciduous forest) of India, where the forest is situated, makes the vegetation in the area more susceptible to ignition and can fuel the spread of wildfires. The region is so dry that several animals die of thirst every year.
● Rising temperatures and changing weather patterns are making the forest more susceptible to fires and can also exacerbate the impact of fires once they start. Generally, the temperature during summers rises up to 50 degrees Celsius in the region, with precipitation as low as approx. 750 mm. Presence of fire affinity invasive species such as Lantana camara sp., Eupatorium sp., propel spread of wildfire.
● Based on our continuous engagement with the Forest Officers and community, most of the reported wildfires are man-made.
● Fires have become a regular occurrence in these dry deciduous forests from November to mid-June every year with a peak in the months of March and April. Some of the man-made activities that contribute to fires in the forest are:
o Encroachment, settlements and human activities in the area, such as slash-and-burn agriculture, uncontrolled campfires and unauthorised logging, lead to accidental fires that can spread quickly and cause significant damage. Lighting fire in the forest is a publishable offence by law, however, in most cases it is difficult to identify culprits.
o Traditional agriculture and foraging practices:
▪ The tribal communities living in the forest collect flowers from Mahua or Butter Tree (Madhuca longifolia sp.) for preparing alcoholic drinks with medicinal properties. The liquor produced from the flowers is largely colourless, opaque and not very strong. It is inexpensive and the production is largely done in home distils. The trees flower in the months of March and April. The mahua flower looks nothing like a typical flower and is almost grapelike – small, round, yellow when ripe, and very sweet – the only giveaways are the pollen and hints of white petals at the top. Therefore, it is really difficult to collect the fallen flowers on piles of dry leaves. The Chenchu tribal community clears the ground by lighting fire on the dry leaves, resulting in a clear, charred-black ground, where the flowers drop from the 20-meter-high canopies. This makes it easy for women of the community to gather the white petaled flowers. A long-standing traditional practice often leads to uncontrolled fires. In response to this recurring issue, the forest department has implemented certain measures to curb these activities. Controlled burning of specific areas and the installation of protective nets around canopy trees are among the strategies employed. Despite these efforts, the challenge lies in the scalability of these measures to address a larger population and a broader geographic area.
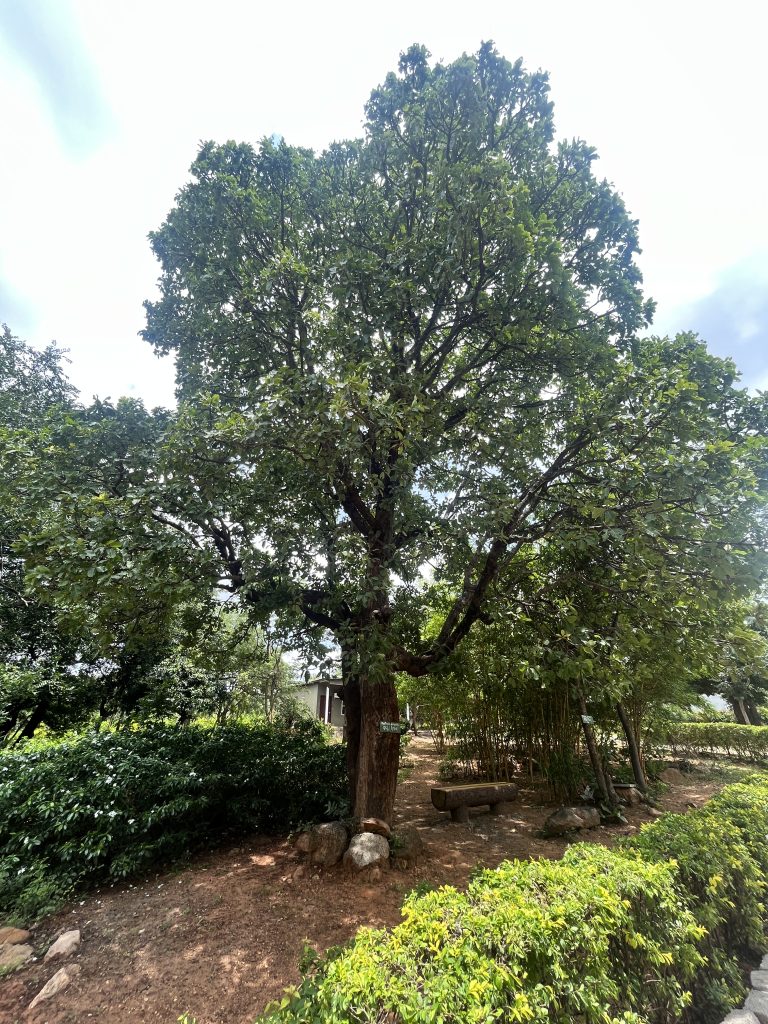
▪ Similar practices are followed for gathering tuber roots. These roots are found in rocky terrains with long thick grasses. Lighting fire helps in clearing the ground. This practice is also followed by illegal poachers.
▪ Additionally, cattle grazers believe that burning grasses leads to greener flush for their livestock to feed on. These traditional activities also lead to uncontrolled spreading of fire. The forest officials have been working on creating fire lines between agriculture land and forest land, but the efforts have not been fully achieved, with only 45% of the total fire lines laid so far to manage the vast forest. The problem is further enhanced due to encroachment farming within the forest.
o Pilgrimage related activities: The pilgrimage to the Srisailam temple, a venerable and ancient religious site in South India, unfolds a unique challenge within the vicinity of the Amrabad Tiger Reserve (ATR). Spanning a 57 km distance, the route between ATR and the sacred temple is ideally traversed by a road that weaves through the forest. However, adhering to tradition, pilgrims opt to walk to the temple from March to April. In their quest to finding the shortest path, pilgrims navigate through the dense forest, where the overgrown grasses make it nearly impossible to locate a path for walking. Consequently, pilgrims’ resort to burning a trail, inadvertently contributing to the risk of causing wildfires. Some people report that they are “helping” other pilgrims to find and travel through the forest easily and counted as “good deeds”. Adding to the complexity, pilgrims often stay within the forest during this pilgrimage, relying on fire for cooking. The improper handling of these fires become a significant factor contributing to wildfire incidents during these months. Furthermore, the fires set by pilgrims are also their way of keeping animals away. These practices are reflective of the complexity between indigenous cultural practices and environmental impact. Effectively addressing these scenarios requires a delicate balance between preserving cultural traditions and implementing measures to mitigate the associated risks of wildfires within the ATR.
The State Forest Department is well aware of the challenges posed by limited resources. In response, the leadership team recognizes the imperative to leverage technology and strategic practices to mitigate wildfire threats and preserve nature. The Department is led by dynamic government officials with background in engineering, technology and previous experience of having worked in global consulting firms. Some of the key strategic initiatives undertaken by them include:
● Identification of the causes for fire
● Fire risk zones identification
● Preventive methods
● Firefighting
● Publicity and awareness
● Record maintenance of areas affected by fire repeatedly through fire control journals
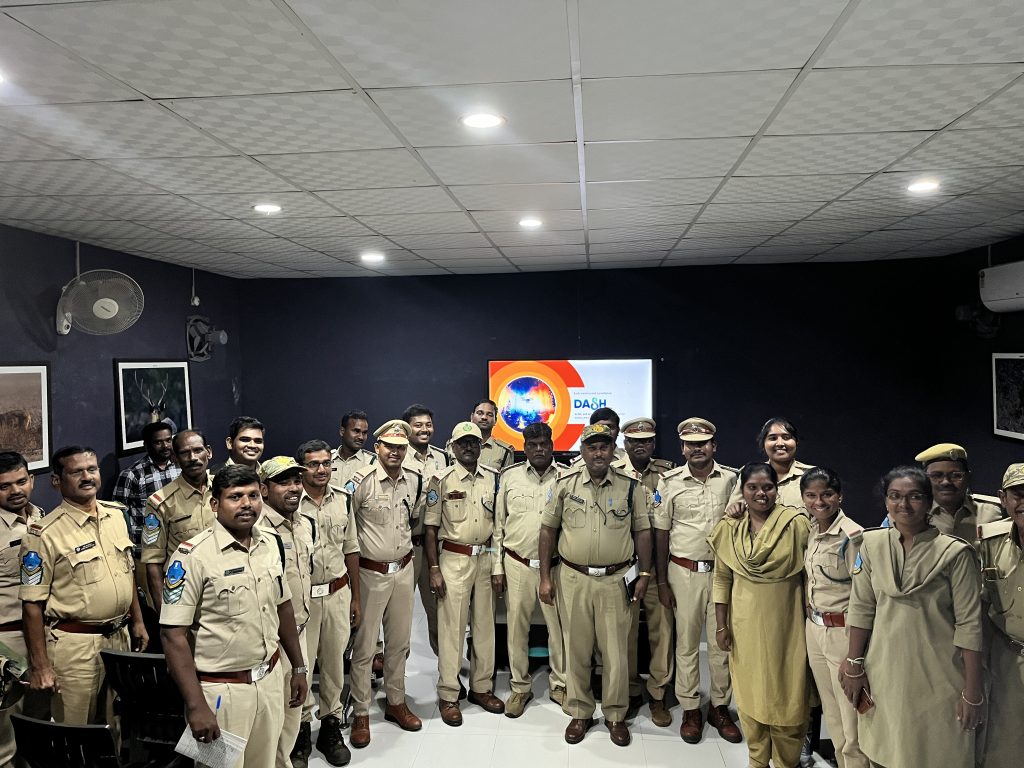
Currently, the forest department is using FSI wildfire alert system, but there are multiple ground level challenges. Here are some use case scenarios:
Example 1: A beat officer starts receiving SMS alerts as early as 2-3 am in the morning (based on the satellite triggers). Upon receiving such notifications, they try to swiftly respond using geo-coordinates. Some report that the incidences have a lag time of anywhere between 6 to 12 hours. Ground staff often consider these alerts as “good to know” but these are hardly accurate or reliable or in-time.
Example 2: When a substantial number of alerts are received in a single day, beat officers leverage their landscape awareness to prioritize responding to fires, particularly if they are located in the dry agricultural areas. These are based on judgement calls only.
Example 3: Some areas are challenging to access and may take up to 4 hours to reach, even to check. Forest officers are able to only assess the situation once they reach the site and then take appropriate actions wherever possible.
The department has identified that there is a need to have a prediction and detection system that can support the beat officers and fire watchers (contracted community/seasonal staffs supporting wildfire mitigation measures). In the words of a beat officer, “…it is heart breaking to see the forest die and nature breaks my willpower to keep on fighting against odds… there are times when we just cannot do anything when the fire starts engulfing its surroundings.”
As part of our pilot, we conducted a range of activities that are deeply rooted in our foundation of human centred design and agile methodology was used for feedback and inputs.
In this section, we share our implementation approach along with lessons learned during the pilot.
Activity 1: Set up the technical working group.
The project commenced with an inception meeting engaging key stakeholders and setting up of the Technical Working Group. This inclusive approach involved orienting pivotal forest department personnel, such as District Forest Officers (DFOs), Forest Divisional Officers (FDOs), Rangers, Beat Officers and Community Engagement Officials and our team members. We were also supported by our Knowledge Partners, the Centre for Wildfire Research (CWR) at Swansea University, United Kingdom, for technical research and knowledge-sharing.
Subsequently, we conducted secondary and primary research to understand our pilot site and audience better. This included understanding about the forest department and its operations, organization structure, role and responsibilities in the chain of command, forest division categorizations, tribal community practices and identifying critical challenges. These constituted the baseline research.
With the guidance of the Technical Working Group, we reviewed existing processes, control room set up, assessed current technologies used, for example, trap cameras to protect wildlife, understand critical factors that influence occurrence of wildfire, land distribution (forest land and agricultural land), landcover, topography etc.
We worked with the Technical Working Group to continuously improve on our AI/ML model to detect and predict wildfires effectively and also tested use cases for drones, including image capture for land use (agriculture vs. non-agricultural) and water-based mitigation measures.
We conducted one-on-one interactions with key stakeholders to understand their roles and also their motivations, learn about the challenges and successes of their everyday work.
Extensive secondary research was conducted to assess regulatory frameworks and perform a technical audit of information and datasets.
Activity 2: Set-up wildfire detection and prediction on site and improved during the pilot.
We conducted real-world testing of the DASH unified system and under the grant. Our aim was to pilot and test this system on the ground to improve it further.
The DASH unified system’s AI/ML model uses diverse datasets, including MODIS and VIIRS satellite data from NASA. Training datasets included fire incident locations, weather/climate data from ECMWF and land cover data from NASA. Land cover datasets include MODIS-based MCD12Q1 data and vegetation indicators like EVI and NDVI. The system was supported with on-ground detection devices and mobile application for reporting. The AI/ML was further enhanced to improve prediction and detection capabilities by integrating a wide range of datasets.
The pilot deployment involved comprehensive site mapping and the installation of various hardware devices, including cameras, sensors, solar panels, and LoRaWAN MESH infrastructure, to facilitate device interconnectivity and data transfer to the platform. The testing covered a total area of 5 square kilometers, equipped with 500 CO2 sensors, 3 cameras, wiring and cables, a processing unit, and mounts or stands. Additionally, portable generators and solar batteries/panels were procured for on-ground wildfire detection. The ground detection system used cameras and IoT devices to monitor soil moisture content, temperature, PPM, and carbon emissions, which were critical indicators for detecting fires in the surrounding area. The MESH infrastructure transmitted this data for analysis. Upon detecting controlled test fires, the system sent notifications to the respective beat officers. We also leased two drones for surveillance and water-based mitigation testing.
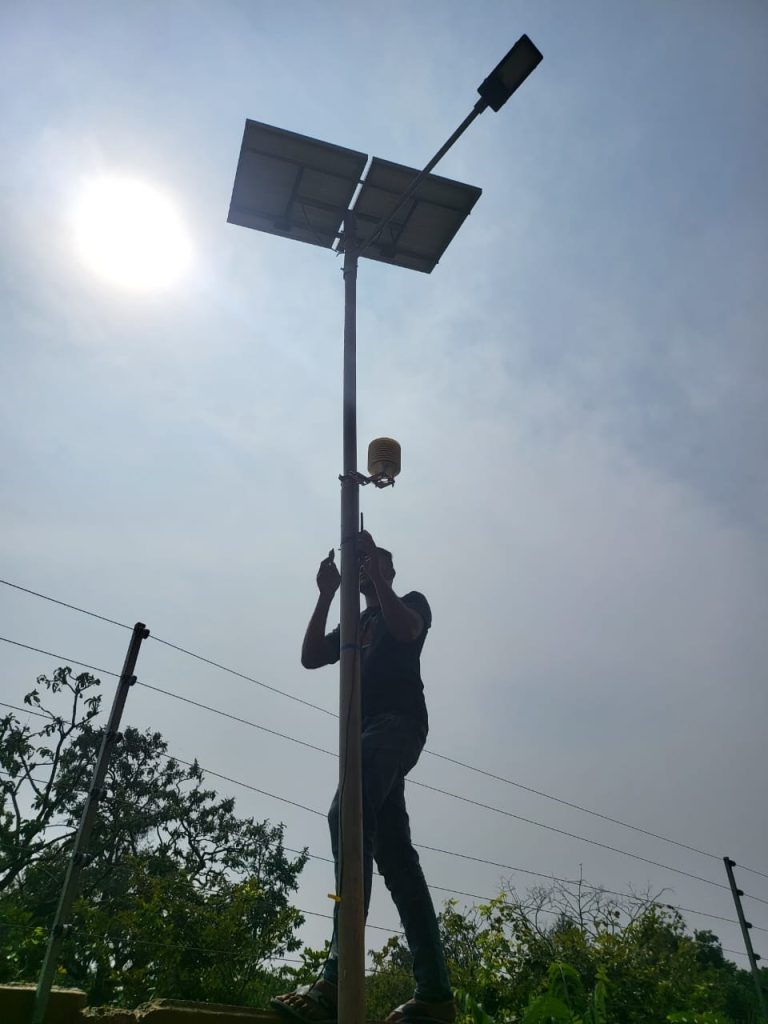
In this phase, we worked through diverse on-ground challenges such as: Is the pole for the camera too heavy to stand straight? Can the pole support the solar panel and the camera together? Is the MESH working adequately? Will the monkeys come and steal the camera? Is the camera setting providing a clear 360 view? We were able to address these and our DASH system started to get feeds and improving its AI/ML model.
We then started to systematically introduce diverse datasets into DASH, including historical data, climatic data (temperature, humidity, wind speed), sensor data, PPM testing results, land cover classifications (encompassing forest and agricultural lands) and shape files. These datasets were pivotal in enhancing the AI-model’s predictive capabilities for wildfire detection and notification to stakeholders. Additionally, our mobile application was customized to facilitate online and offline reporting of wildfire instances, incorporating features such as photographic evidence capture with timestamp. These were based on additional fields identified in consultation with the beat officers and forest officers.
We have been continually learning and working on comparing our prediction and detection results with other wildfire data sources such as satellite-based Forest Survey of India (FSI), NASA and actual reporting from the ground. For instance, on 1st February, 2024, there were 4 fires detected in the system and this has been validated at all levels, giving reliability in the detection measures. We also conducted sensor testing to understand thresholds to detect fire from smoke.
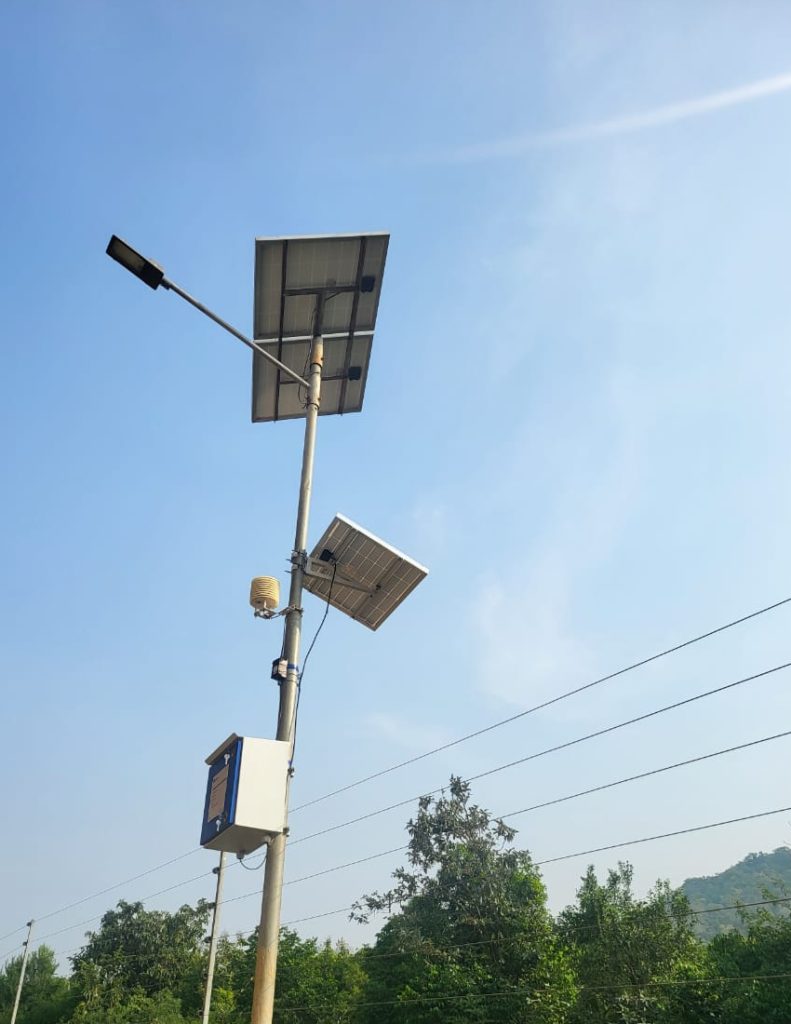
On other occasions as well, the system successfully detected fires, which were validated by on-ground reporting. These occurrences highlight the system’s effectiveness and with effective machine learning, over time, the confidence interval has also improved. In addition to this, we have conducted sensor testing to determine the thresholds required for accurately detecting fire from smoke. These tests were carried out at the testing site.
Moreover, our efforts have resulted in a notable reduction of false positives by 30% thus far. Looking ahead, with the integration of additional datasets and through continuous refinement using machine learning techniques over time, we anticipate further reducing false positives by 80-90%.
It is important to note that we have identified a minimum threshold of 300 sensors to accurately detect fire within a 5 square kilometre area, ensuring optimal coverage and detection efficiency.
As mentioned, the biggest cause of fire in the area is human behaviour. Hence, we started to plan to supplement our AI/ML model with human behaviour patterns. This included the team to brainstorm approaches on training the algorithm on observed wildfire occurrences along specific forest routes and areas. Based on our research, integrating human behaviour patterns to the AI/ML model shall further improve the confidence of reporting and managing of ground resources. Further datasets and processing are required to enable machine learning for even better accuracy and efficiency. Further research and development are also required to ascertain how to incorporate behavioural patterns for behavioural analytics into the system.
Activity 3: Drone deployed for wildfire mitigation.
Wildfire mitigation is an important aspect of strategic wildfire management. We have piloted the use of drones for testing two aspects – surveillance and combating wildfire.
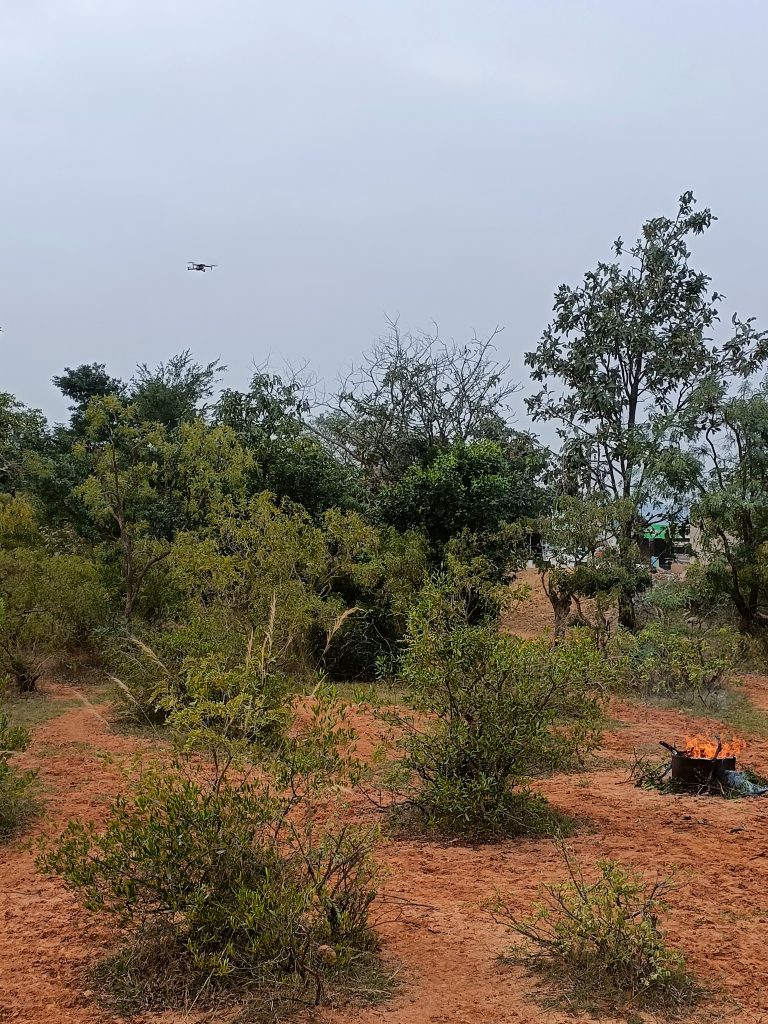
In our discussion with stakeholders from the forest department, we had learnt that due to the vastness of the forest, it is extremely difficult to conduct surveillance, especially for the rocky terrains. When a wildfire is detected, the beat officer, along with fire watchers, are the first responders to reach the site for ocular inspections. Based on this assessment, the back-up teams are initiated or other measures are taken. There is significant loss of time between notifications received and actions taken.
Additionally, when combating wildfires, there are challenges such as:
● Inadequate firefighting gears.
● Hard-to-reach areas due to lack of motorable roads and hilly terrain; it is also time consuming.
● Difficult to assess the wildfire impact coverage without any data; it is mostly based on judgement calls.
● Heavy dependence on the experience of ground staff to assess epicentre, tiggers, path of fire, best course of action for mitigation measures.
● Wildfire management is exhausting as fire remnants may trigger new or fresh fires.
● Safety of first responders is often compromised by fire and wildlife attacks due to panic and trauma (e.g. sloth bears and pythons).
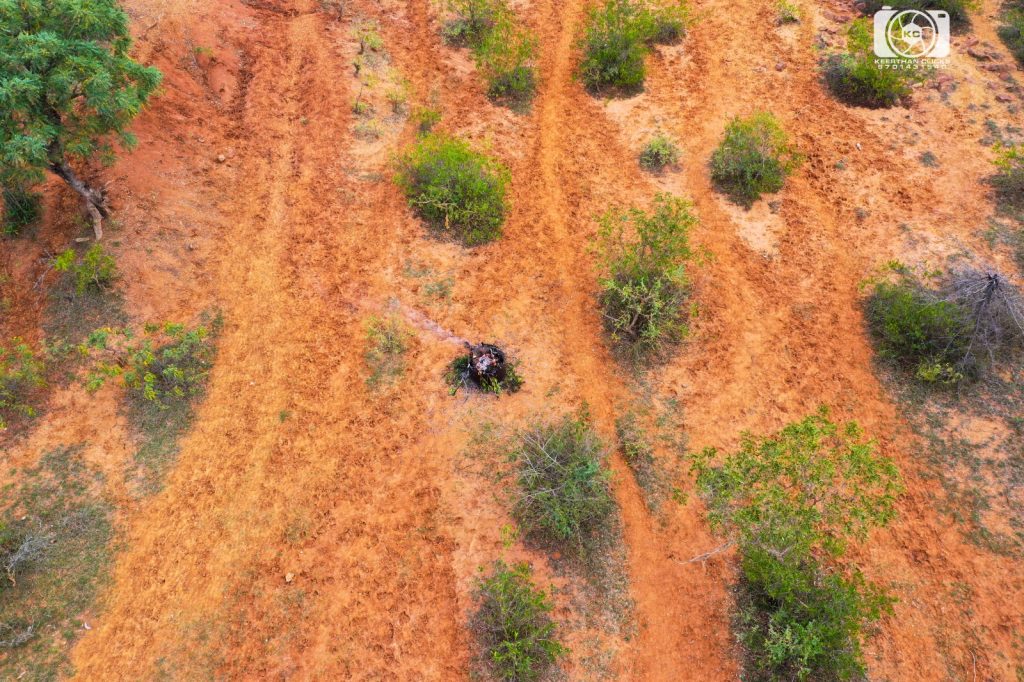
As part of the pilot, drones were utilized for simulation purposes, to gather data and evaluate suitability for regular use in mitigation and post-disaster assessment efforts. The following activities were undertaken:
● We deployed the drone to map land cover accuracy, as current data lacks precision in distinguishing between forested and agricultural areas. This mapping was conducted both during daytime and night-time, in normal conditions, to establish a foundational dataset for training the AI/ML wildfire detection algorithm.
● Drone surveillance was tested using controlled fire scenarios, varying in intensity, to assess data feeds and identify information that could refine the algorithm’s ability to detect wildfire signatures. This phase also involved integrating sensor data collected during drone flights to enhance algorithm training. Testing was conducted at different levels of fire intensity to determine the baseline for fire alerts.
● Drones equipped with water dissemination were tested to evaluate coverage and information relay capabilities, offering insights into wildfire mitigation strategies.
All of these activities were carried out within controlled test environments due to specific guidelines and approval procedures governing drone use in forested areas. Through this process, we gained insights into regulations, compliance and limitations associated with drone operations, such as flight speed, elevation, load capacity, data connectivity, and the use of fire suppression chemicals in forested regions.
During these activities, we systematically conducted controlled fire tests to evaluate the drone’s capabilities. This involved conducting fire detection tests 3-4 times under controlled conditions to assess the drone’s effectiveness in identifying wildfires. Additionally, we performed 3-4 tests to obtain accurate land cover data and establish live data transfer from the drone to the system, crucial for enhancing our understanding of the terrain and improving real-time monitoring capabilities. We also conducted 3-4 tests to evaluate the efficacy of water as a mitigation measure when disseminated via drone. These comprehensive tests allowed us to assess various aspects of the drone’s functionality and its potential contributions to wildfire management efforts.
The drone demonstrated a flight time of 20 minutes per launch, adhering to the limitations set by the Indian Government’s authorizations, with an altitude limit of up to 200 feet. For scaling up operations, special permissions and licenses can be obtained by Government Forest Departments.
Drones were found to be most effective when wind speeds are below 10 knots. Despite current limitations, such as specific clearance requirements for larger drones, the pilot instills confidence in the effective utilization of drones for terrain analysis and fire mitigation in remote areas. For instance, designated tourist spots could benefit from regular drone surveillance and the deployment of water dissemination upon fire detection.
Activity 4: Technical and ground staff trained on using the system effectively.
Every year, from November onwards, refresher training sessions are held for beat officers and fire watchers in anticipation of the wildfire season from December to April in the forest. These sessions take place in physical workshop settings and include safety drills and instructions on equipment usage, particularly blowers. The training primarily emphasizes ground activities and traditional mitigation methods. Despite their familiarity with smartphones and digital devices, no e-learning or technology-based training is currently provided.
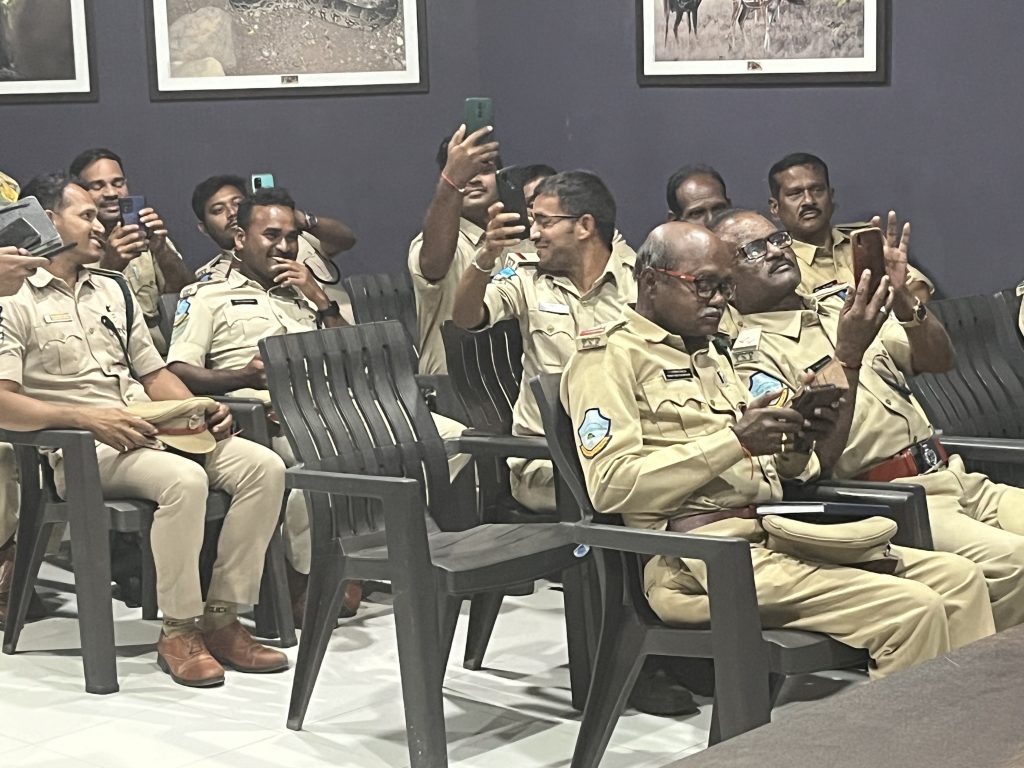
As part of introduction to DASH unified system, a comprehensive training plan has been designed to facilitate the beat officer capacity building process. The training commenced with a technical session conducted for 24 Beat Officers, focusing on the adept utilization of the dashboard and mobile application for seamless reporting. The training focused on equipping beat officers with the skills and understanding necessary to utilize the application effectively. A key component of the training was the proper use of a reporting form designed to be submitted when a fire incident is detected. Through hands-on instruction, officers gained proficiency in navigating the platform’s features and utilizing its functionalities for timely reporting.
Following the technical training, beat officers were onboarded to the DASH system. This demonstrated their familiarity and proficiency with the system’s functionalities. Feedback was collected from the participants. All participants shared that the mobile application was easy to use and they did not face any challenges in navigation. They found the reporting useful and some suggestions were provided for improving the reporting form fields, which were incorporated.
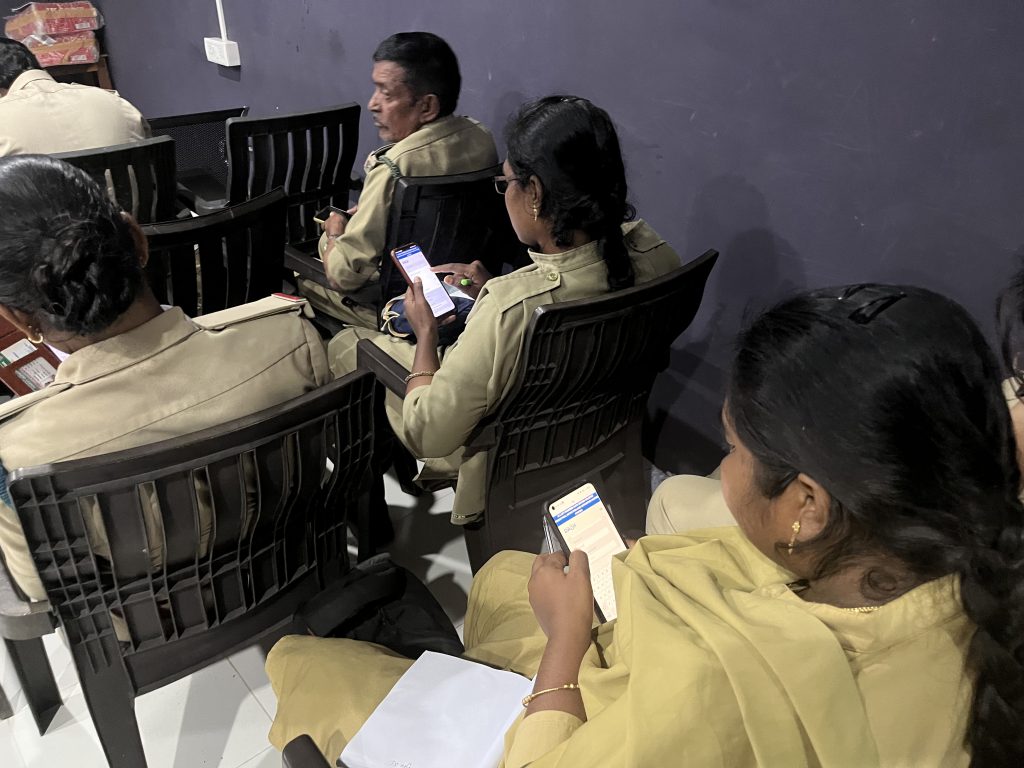
This collaborative and engaging activity not only facilitated the transfer of knowledge but also marked a significant stride towards inclusivity, with the participation of 6 women beat officers in the training sessions. We planned to conduct additional physical training, however, due to organizational priorities and peak wildfire season, these could not be conducted. We also added curated best practice videos on the mobile app for self-paced learning for the officers.
Activity 5: Awareness sessions conducted with community members
Near the pilot vicinity, there are around 17 villages inhabited predominantly by members of the Chenchu tribe, with a total population of approximately 2000. The community members are engaged in cattle rearing, foraging, gathering non-timber-products from the forest etc. We have furthered our understanding of the community, based on continuous engagement with the community members during the course of the pilot implementation.
As prescribed by law, the tribal communities living within the forest are provided with a range of government support services. The overall social and individual mindset of the community is unique, as compared to that of city inhabitants. For instance, they are aware of the city life, the latest smartphones in the market, are literate with basic education but choose to live in the forest due to ancestral linkages, land ownership and complex dependency on government social protection incentives. They are vocal for preserving the forest and consider themselves as guardians of the forest as they find themselves most comfortable staying within the forest and away from the bustling city-life.
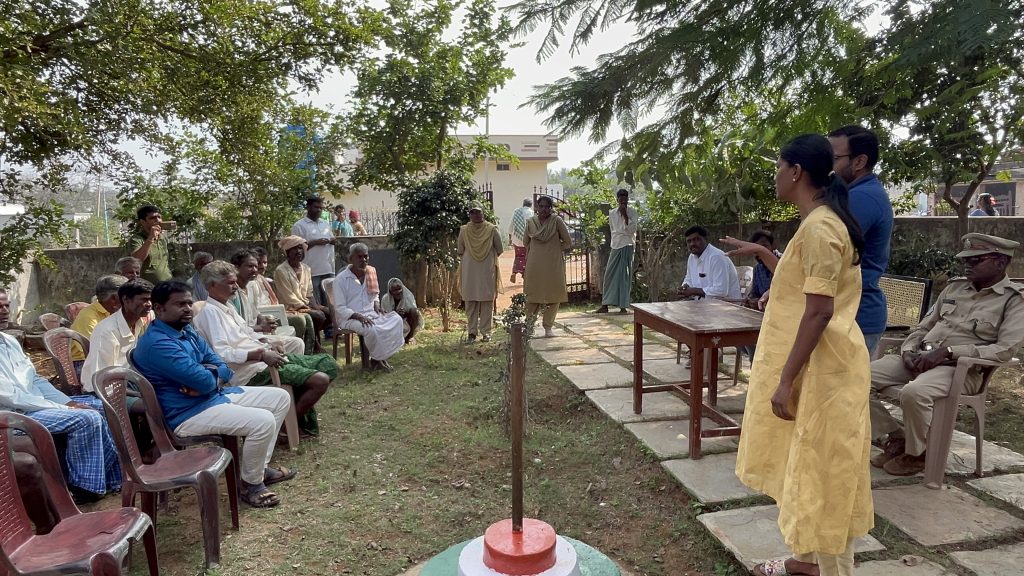
Women are mostly engaged in household activities, flower gathering and child rearing. They have limited role in engaging with outsiders and wildfire management. During the wildfire season, the Forest Department contracts women from the community for clearing out weeds from the forest and to assist in dousing fire by carrying water. Some of the women have also joined the Forest Department’s skills development initiative related to tailoring of jute bags that come with customize branding and awareness messages for forest protection. Others are also involved in supporting farming in apiculture and handicrafts.
Two local members were designated as trainers to lead community awareness sessions conducted in the native language. The design of these training sessions was done in a way to effectively engage with the community, focusing on discussing best practices for wildfire prevention and providing support in mitigation activities in the event of wildfires. In our conversations with the community, it was also evident that some had awareness on the challenges of wildfire, while others consider fires as necessary for nature’s balance.
Through these engagements, we identified the need for integration of social media for community members to be empowered to play an active role in the detection process. In this scenario, if a community member identified a fire, they would promptly report it through the social media group. This user-generated report was seamlessly fed into the system, triggering an automatic detection alert. This innovative approach not only harnessed the local knowledge and awareness of community members, but also ensured a swift response to mitigating the fire at its earliest stage. The Telegram-bot was designed and tested in a small test scenario. Interactions with community also revealed that there may be need for WhatsApp or SMS-based integration.
Our team interacted with the visiting tourists and designed a short playful awareness session with children and guardians on the importance of preserving forest and behavioural do’s and don’ts. We conducted additional training session with forest camp care-takers and jeep-drivers. This was to ensure that as part of their tour and interaction with the tourists, they were able to talk about wildfire and associated challenges.
Total number of participants for training as part of the pilot included:
· Technical Working Group = 5
· Beat Officers = 24
· Forest department contract staff from community (care-takers, social media manager, trainer, outreach officers) = 10
· Community training = 35
· Overall engagement = 74
Activity 6: Partnerships established for enhancing the impact
Our project is exploring partnerships with other organizations, researchers and community leaders. Collaborative efforts are facilitating data sharing, research collaboration and community engagement initiatives, strengthening the impact and reach of our wildfire management efforts.
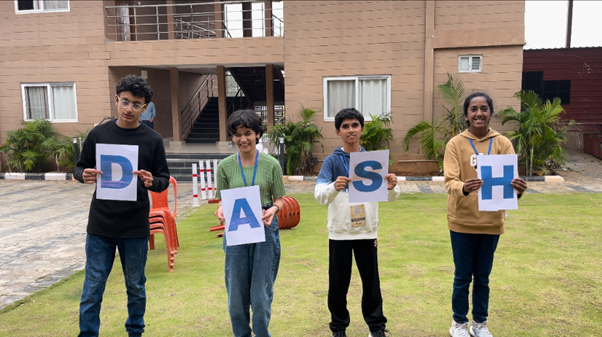
We have received positive feedback from the Forest Department regarding the system’s effectiveness in detecting and predicting wildfires. Ongoing discussions have led to requests for improvements in the predictive capabilities, particularly by incorporating human behaviour datasets.
We were invited by UNESCO to present the DASH unified system at the Ridge to Reef initiative organised in Chennai, in collaboration with the Ministry of Environment, Forest and Climate Change of India. Heads of Forest Departments and Technology Unit officials who attended our session appreciated the innovation.
We have received inquiry and potential interest from four other State Forest Departments (Himachal Pradesh, Uttarakhand, Chhattisgarh and Kerala) in exploring DASH unified system’s potential for their forests, with discussions ongoing.
Our team visited Shimla, Himachal Pradesh and Thiruvananthapuram, Kerala, to demonstrate the system to the State Department of Forest officials and also gather initial baseline data and exploring areas for implementation of the system. We initiated discussions to explore opportunities in Uttarakhand, as the State prepares to take steps towards disaster preparedness. Uttarakhand is a Himalayan region mountain state, that is regularly impacted by wildfires and other natural disasters. The Government of Uttarakhand has developed a comprehensive institutional set-up to address climate and disaster risks, but gaps remain in the State’s emergency preparedness.
As part of the interactions with development partners such as the World Bank and UNDP, it has emerged that there is a need for unified systems such as DASH, that are easily scalable and have comprehensive implementation approaches that bring together all stakeholders. There is a need to leverage the platform across different use cases for effective utilization of resource. For example, the reporting application may be used for sending reports not only on wildfire but other wildlife risks (poaching evidences, human-animal conflict, illegal logging etc.) and also use comprehensive dashboard based on indicators and roles.
Project Activities, Deliverables and Indicators
Activities
Beginning of project
Activity | Description | #Months |
---|---|---|
Inception and initial set-up | ● Conduct inception meeting with key stakeholders to identify and set up a Technical Committee and Working Group. ● Identify current practices and challenges, zoning matrix and conduct baseline research. ● Provide access to DASH AI and IoT Powered Data Driven Wildfire Detection and Prediction System and set up Amrabad Tiger Reserve visualisation dashboards and alert notifications. ● Conduct training of technical staff for using the dashboards and reports. ● Co-create and identify specific integration for on ground wildfire management using IoT. | 1 |
Middle of project
Activity | Description | #Months |
---|---|---|
Pilot implementation | ● Conduct training of on-ground staff in using DASH - login, access information, submit reports, document images and using it as a learning tool. ● Support in awareness training for community response towards preventive and mitigation measures. ● Regular monitoring, training, testing and validating the wildfire AI-model with additional and live datasets. ● Work closely with Amrabad Tiger Reserve team in their efforts to mitigate wildfires. ● Integration of drone and its deployment when required for surveillance and wildfire mitigation. ● Document complete report on events with onground verification and feedback. ● Collect continuous feedback on useability, accessibility, reliability, accuracy of the system. | 4 |
End of project
Activity | Description | #Months |
---|---|---|
Prepare detailed report and communication materials | ● Conduct evaluation, gather feedback, conduct technical analysis, prepare project report and recommendation for scaled operations ● Create communication materials for dissemination and awareness. | 1 |
Deliverables
Deliverable | Status |
---|---|
Set up technical working group within 2 weeks of project commencement and ensured active participation. | Completed |
Set up wildfire detection and prediction on site and improved during the pilot. | Completed |
Drone deployed for wildfire mitigation. | Completed |
Technical and ground staff trained on using the system effectively. | Completed |
Awareness sessions conducted with community members. | Completed |
Partnership(s) established for enhancing impact. | Completed |
Key Deliverables - Detail
Deliverable: Set up technical working group within 2 weeks of project commencement and ensured active participation. Status: Completed Start Date: November 7, 2023 Completion Date: November 18, 2023 Baseline:There is neither a dedicated team overseeing nor a structured process for wildfire data. A technical working group shall play an important role for enabling setting up customization in the system and providing inputs throughout the pilot. Activities: 1. Initiate an inception meeting with key stakeholders to identify objectives and establish a Technical Working Group. 2. Orient key representatives from the forest department, such as DFO, FDO, Rangers, Beat Officers and the community, to participate in the Technical Working Group. Additional members comprise our Knowledge Partners and team members. 3. Identify prevailing practices, challenges, zoning matrices etc. 4. Undertake thorough secondary research to analyze regulatory guidelines and perform technical audits of information and datasets. Outcomes: Set up of technical working group. Facilitating effective information gathering, maintaining stakeholder communication and establishing an efficient feedback mechanism. Additional Comments: Establish the technical working group with a minimum of 5 members across key stakeholder categories within the initial two weeks of the project's initiation to ensure their involvement from the outset. |
Deliverable: Set up wildfire detection and prediction on site and improved during the pilot. Status: Completed Start Date: November 28, 2023 Completion Date: March 28, 2024 Baseline:Wildfire is recognized as one of the major concerns and even though there is no structured process, the following are the current ad-hoc practices for wildfire detection and prevention: • Physical watch tower guarding, which is not efficient as most towers remain unmanned due to harsh summers and human fatigue. • Satellite data via Forest Survey of India's Fire Alert System (https://fsiforestfire.gov.in) for Detection and Early Warning. Forest Survey of India advises all forests to use this data in conjunction with other sources of forest fire detection and not solely rely on these satellite based fire alerts for tactical firefighting. • Recruit seasonal staff from villages as “Fire Watchers”. Additional contract staffs are recruited for clearing of weeds from the forest (lantana camara and phoenix plants) prior to the summer months. Currently, section and beat officers receive wildfire alerts through two primary channels: (a) SMS notifications from FSI alert system and (b) direct emergency calls initiated by villagers or other watchtower guards. During the dry summer months, a substantial volume of alerts (approximately 10,000) are received by beat officers, making it difficult to manage. Several challenges are associated with these alerts, including significant delays in their reception (typically 10-12 hours lag time), instances of ground-level reporting preceding SMS notifications, limited accuracy, a heavy dependence on manual sorting of fires that should be responded to urgently and identifying the terrain or movemement for those fires. Forest Fire Alert System provide early warning (1 month advance prediction of wildfires) based on trends of the same satellite data. There is no AI/ML being used for the prediction. Ground staff indicate that these predictions are “good to know“ but are hardly accurate or reliable. Here are some illustrated cases for detection: Example 1: A beat officer starts receiving SMS alerts as early as 2-3 am in the morning. Upon receiving such notifications, they try to swiftly respond and are dispatched to wildfire locations using geo-coordinates. Some areas are challenging to access and may take up to 4 hours to reach. Forest officers are able to only assess the situation once they are on site and then take appropriate actions wherever possible. Example 2: When a substantial number of alerts are received in a single day, beat officers leverage their landscape awareness to prioritize responding to fires, particularly if they are located in the dry agricultural areas. These are based on judgement calls only. Activities: 1. Initial deployment of the DASH unified system, including site mapping and setup of hardware devices such as cameras, sensors, drones, solar panels and LoRaWAN MESH. 2. Gradual incorporation of datasets to enhance the AI model, including historical data, climatic data (temperature, humidity, wind speed), sensor data, PPM testing results, land cover classifications (encompassing forest and agricultural lands) and shape file, aiming to predict, detect and notify stakeholders about potential wildfire incidents. 3. Tailoring of the mobile application with online and offline functionalities, including reporting of wildfire occurrences, allowing for the submission of photographic evidence, documentation of burn areas, and assessments of biodiversity loss. 4. Advanced training of the AI model by integrating human behavioural patterns observed in specific routes and areas of the forest, recognizing the critical role of such data in predicting and preventing man-made wildfires. 5. Validating the systems with benchmarking and triangulation of information. Outcomes: The algorithm has undergone continuous training and refinement, leveraging ongoing machine learning techniques to optimize its performance. As a result, significant improvements have been achieved in predictions. This enhancement has been made possible by incorporating additional datasets, enabling faster detection of wildfires. The DASH unified system facilitates the ongoing integration of new datasets, beyond the ones used for the pilot. This is currently being explored with the Indian Meteorological Department. The system has also been enhanced with a unique social media reporting and detection mechanism, resulting in a reduction of notification times to less than 2 hours, with ongoing machine learning capable of bringing this down to minutes. Initially, we conducted confidence level checks to refine the system’s accuracy, addressing instances where any type of fire from any zone or district was reported. Through controlled fire testing, we were able to send over 50 notifications throughout the pilot duration, each relevant to a specific area. Notably, no natural fires occurred during the pilot period in our designated area. Furthermore, the system has demonstrated an impressive 50% increase in accuracy rates and a reduction in false positives, marking significant progress in its reliability and effectiveness. Regular system checks were conducted to ensure optimal functionality and performance. Additional Comments: The DASH unified system is set up and is able to detect and predict wildfires. Wildfire detection is improved, including reduced alert time (< 2 hours) is achieved through IoT sensors, cameras and diverse data sets, increasing accuracy by minimising false positives and providing a unified dashboard for alerts and reporting. AI-model is continually trained and improved for prediction of wildfires by pattern recognition, hot-spot mapping using diverse datasets such as satellite, historical data, landcover, weather, windspeed, habitation etc., as well as data from the ground. |
Deliverable: Drone deployed for wildfire mitigation. Status: Completed Start Date: January 5, 2024 Completion Date: March 28, 2024 Baseline:When a wildfire is detected, beat officer along with fire watchers, are the first responders to reach the site for ocular inspections. Based on this assessment, the back-up teams are initiated or other meaures are taken. There is significant loss of time between notification received and actions. Some of the other challenges include: • Time consuming and hard to reach areas due to lack of motorable roads and hilly terrain. • Difficult to assess the wildfire impact coverage without any data; mostly based on judgement calls. • Heavy dependence on the experience of ground staff to assess epicentre, tiggers, path of fire, best course of action for mitigation measures. • Wildfire management is exhausting as fire remanents may tigger fresh fires. • Safety of first responders is often compromised by fire and wildlife attacks due to panic and trauma (e.g. sloth bears and pythons). Activities: 1. Use drones to map landscapes under different conditions for training AI/ML wildfire detection algorithms. 2. Test drone with water sprinklers as one of the measures for wildfire mitigation. Outcomes: During our pilot project, we explored the integration of drone testing for surveillance and assessed its effectiveness in mitigating wildfire incidents. The strategic deployment of drones proved instrumental in enhancing wildfire detection efficiency and monitoring capabilities. With the ability to cover up to 2 square kilometers in a single flight, drones proved effective for small-scale fire incidents and provided small-range coverage essential for timely response. In the pilot area spanning 5 square kilometres, there were no natural fire incidents during our testing period. Controlled fire testing demonstrated significantly faster response times compared to traditional methods, showcasing the operational advantages of utilizing drones in fire mitigation efforts. Through the utilization of drones, improved response times and enhanced capabilities in mitigating fire incidents is possible, thereby contributing to more effective wildfire management strategies. Additional Comments: The DASH unified system uses drones for terrain mapping and assessment of wildfires and provides assistance to the first responders, leading to reduced response time, identifying patterns and threats, conducting heat surveillance etc. before teams are dispatched on-site. |
Deliverable: Technical and ground staff trained on using the system effectively. Status: Completed Start Date: December 11, 2023 Completion Date: March 15, 2024 Baseline:Refresher trainings are conducted for beat officers and fire watchers in the month of November every year as a preparation for December to April wildfire season in the forest. The training is done in small physical workshop setting and covers safety drills and use of the equipment (blowers). The beat officers have a Bachelor’s degree and fire watchers have basic literacy in vernacular language (Telugu). The training is focused on ground activities and traditional mitigation measures. Even though they are comfortable with using smartphones and other digital devices, no e-learning or tech-based training is being conducted. Activities: 1. Designed training plan for the beat officers’ training. 2. Conducted technical training for 24 Beat Officers on use of dashboard and mobile application for reporting. 3. Onboarded beat officers on the DASH platform. Outcomes: Successfully trained and onboarded 24 beat officers, enabling them to download, login and practice reporting using the application, with positive feedback received. Additional Comments: Conduct training with a total of 10 technical and ground staff within 2 months of project initiation and measure impact through post-training assessment and continuous feedback during the pilot. A. Post training assessment (knowledge and practice) scores above 90%. B. Training feedback. C. User feedback survey on pilot. |
Deliverable: Awareness sessions conducted with community members. Status: Completed Start Date: December 11, 2023 Completion Date: March 15, 2024 Baseline:Near the pilot site, there are around 17 villages inhabited predominantly by members of the Chenchu tribe, with a total population of ~ 2000 (70-150 people per village). The villagers are engaged in cattle rearing, foraging, gathering non-timber-products from the forest etc. Some of these activities lead to clearing forest lands using fire. According to the villagers, forest fires are traditional ways of living and part of the natural balance. The Forest Department has been conducting awareness programmes at the village level. However, the training is unstructured. Women are mostly engaged in household activities, flower gathering and child rearing. They have limited role in engaging with outsiders and wildfire management. During the season, the Forest Department contracts women from the community for clearing out weeds from the forest and to assist in dousing fire by carrying water. Activities: 1. Identified local staff to lead community awareness sessions. 2. Designed and scheduled training sessions. 3. Conducted awareness training sessions at the village level. 4. Designed short playful awareness sessions for tourists (children and guardians). 5. Designed approaches for social integration including Telegram and social media. Outcomes: Equipped 35 community members with skills for collaboratively working with the forest department in wildfire management. Additional Comments: Conduct awareness sessions with a total of 30 community members (at least 80% ethnic minority, up to 40-50% women, 10% individuals with special needs), within 4 months of project initiation and measures impact using attendance sheets and feedback tools. |
Deliverable: Partnership(s) established for enhancing impact. Status: Completed Start Date: November 2, 2023 Completion Date: April 27, 2024 Baseline:Currently there is no partnership established. Activities: 1. Interacted with wider stakeholders in different events to present DASH and its pilot activities. 2. Provided demonstration to key stakeholders from the forest department as well as other Government and development partners to garner support and enhance project’s impact. Outcomes: Work in progress to sign MoUs with forest departments and other stakeholders such as the Indian Meteorological Department. Additional Comments: Formalize and sign at least 1 Memorandum of Understanding (MOU) within the 6 months of the project, with authorities, firefighting agencies or other relevant organizations to enhance the project's impact. |
Project Review and Assessment
The DASH unified system, as an innovative solution, has the potential to provide technology - enabled strategic implementation that has the potential to make wildfire management more effective and efficient.
It aligns to the strategic vision of the State Forest Department to leverage technology to assist the on-ground staff in directing their efforts and resources towards protecting the forest. Achieving its primary objectives, DASH was piloted in the real-world for predicting and detecting wildfire alerts in real-time, thereby minimising risks associated with delayed responses. The system’s backbone consists of a lightweight cloud platform and advanced AI/ML models to predict, detect and mitigate wildfire events. The AI/ML model is trained on diverse datasets like satellite imagery, landcover type, climatic dataset and sensor data, enhancing the system’s predictive accuracy and adaptability.
The DASH system supports in Internet development, through creating a case for using technology for community and environment. For example, we have set up LoRaWAN MESH infrastructure for our devices to interconnect and transfer data to our platform.
DASH also utilises IoT technology for comprehensive environmental monitoring and employs a modular approach for adaptability. The ongoing work on integration with social media and a mobile app for real-time updates represents the project’s effective use of Internet infrastructure for communication and response. Looking ahead, the project holds substantial potential for growth, envisioning an expansion into various environmental and forest monitoring domains, thereby evolving into a more holistic system.
The platform’s user-friendly interface and data-driven dashboards offer an easy-to-understand, holistic view of the wildfire landscape. These features not only facilitate environmental monitoring but also empower authorities with timely and actionable insights. The incorporation of self-guided learning resources and a robust offline mobile application have enhanced the system’s capability to assist stakeholders in effective disaster management. DASH’s adaptability is further exemplified by its validation for customization across different geographies with minimal infrastructure investment, showcasing its potential as a versatile solution.
The system’s practical effectiveness is demonstrated by its capacity to detect live fire incidents, with ongoing improvements and technological innovations continuously being integrated based on feedback.
Support for local technical capacity is a cornerstone of the project, with comprehensive training sessions for beat officers and collaborations with forest officials for community outreach, ensuring effective use and real-time reporting capabilities.
The lessons learned highlight the importance of implementing human centred and agile development methodology, on-ground research, technical training, community engagement and a modular approach for successful project development. Through these initiatives, DASH not only facilitates capacity building among institutions and individuals, but also sets a precedence in project design, management and implementation, by incorporating behavioural research and creating a robust notification system.
The following are the key strengths of the pilot and DASH unified system:
Comprehensive Unified Approach: The project takes a comprehensive approach to wildfire management, integrating AI/ML algorithms, IoT sensors, mobile application and community engagement strategies.
Technological Innovation: Leveraging advanced technologies like AI/ML and IoT for wildfire prediction and detection demonstrates innovation and potential for effective solutions. It also involves integrating mitigation measures that can help save lives of first responders, tracking and diminishing chances of reignition and protecting the forest health overall.
Cost-Efficient: Leveraging AI/ML models, the system effectively enhances prediction and detection through economical approaches compared to alternative solutions. The cost of similar systems varies depending on implementation modalities. However, the DASH unified system is specifically designed to tackle challenges encountered by many developing countries’ forest departments, accommodating existing solutions and facilitating subsequent scalability.
This unified system allows for the incorporation of additional datasets to tailor the AI/ML algorithms, aiding in customization and enabling seamless integration for broader forest information management, thus creating a comprehensive one-stop solution. Currently, the forest departments rely solely on satellite detection and manual methods, often taking between 6-12 hours, while detection takes less than 2 hours using the DASH system. With continuous machine learning, the system is geared towards detection time being reduced to minutes.
DASH system is lightweight, cloud-based, with low processing costs, facilitating effective deployment, training and maintenance. This contrasts with the current model, where technology systems are absent or limited in their capabilities and performance.
Other solutions in the market are not unified and often specialize either in prediction or detection alone. Their implementation costs per coverage area are significantly higher, often multi-fold, compared to the DASH system. By leveraging cloud-based processing, the DASH system significantly reduces hardware costs and facilitates quicker implementation, thereby making forest management more cost-effective and efficient.
Stakeholder Engagement: The project actively engages with key stakeholders, including forest departments, local communities and environmental organizations, fostering collaboration and ownership.
Pilot Implementation: Conducting a pilot implementation in the Nallamala Forest area provides real-world testing and validation, allowing for iterative improvements and learning.
Partnerships and Recognition: Establishing partnerships with other organizations and receiving interest from state forest departments demonstrates credibility and potential for scalability.
The following are the key learnings and areas of improvement from the pilot of DASH unified system:
Using Human Behaviour for AI/ML model: At the pilot site, there are multiple human behaviours, such as agricultural practices and pilgrimage rituals that have previously triggered massive spread of wildfires. Every year during the pilgrimage season, the forest is dotted with fire hotspots. We conducted initial research to understand the human behavioural patterns and further research and development on how to incorporate this data for behavioural analytics to further enhance the DASH system.
Regulatory and Compliance: As part of our inception and technical set up period, we reviewed and also consulted with the forest officers regarding compliance with regulations related to forest land area access and restrictions related to fire testing, drone usage, data privacy and environmental impact assessments etc. These regulatory and compliance measures also need to be considered for the scale-up and sustainability of the system.
Communication and Awareness: Human behaviour is one of the key causes of wildfire in the forest. The practices are deeply embedded in social and cultural contexts. It is critical to ensure that for effective communication and awareness among stakeholders, especially in rural and remote communities, targeted strategies and ongoing engagement efforts are required to maximize impact and participation. Additionally, responsible tourism awareness programmes need to be emphasised.
In essence, the DASH project stands as a testament to the power of technological innovation, strategic planning and community involvement in addressing critical environmental challenges. Its multifaceted approach not only addresses current wildfire management needs but also lays a foundation for future advancements in environmental monitoring and disaster management.
Diversity and Inclusion
The project has prioritized and actively incorporated diversity and inclusivity as integral elements of its implementation strategy. In addressing gender inclusivity, efforts have been made to involve women in various aspects, including technical training for beat officers. The training included women beat officers and community workers.
To address cultural and language diversity, the project engaged members proficient in the local dialect for community outreach. This proactive measure facilitated effective communication during awareness sessions. Additionally, the project recruited local staff for ground research in behavioural studies, ensuring that language differences did not impede the collection of valuable data.
Acknowledging cultural constraints that restricted women’s participation in community activities, the project implementation acknowledged the need for innovative approaches. The experience also highlighted the complexity of achieving perfect inclusion rates across diverse stakeholder and beneficiary groups, leading to a more nuanced and adaptable approach in community engagement.
Our team members come from diverse backgrounds and possess a range of skills and expertise, culture, language and perspectives. This diversity enriches our ability to develop innovative and inclusive solutions that are responsive to the needs of diverse communities. These initiatives collectively underscore the project’s commitment to embracing diversity and fostering an inclusive environment both within the organization and the community served.
Project Communication
The project’s communication strategy unfolded through strategic steps aimed at fostering collaboration, raising awareness and sharing advancements. The establishment of a Technical Working Group, comprising of the DFO, forest rangers and beat officers, marked a crucial activity. This collaboration not only provided insights into the Department’s vision, but also of specific on-ground realities and challenges. It also facilitated gathering necessary permissions to conducting the pilot.
The deployment of the DASH unified system and continual improvements on analytics are an ongoing activity, based on the ongoing communication with key stakeholders, followed by targeted training sessions for beat officers on application usage. Simultaneously, partnerships with forest officials engaged in community outreach addressed language barriers, enabling awareness sessions in the local dialect. This engagement, however, posed challenges in conducting ground research for the platform’s behavioural research component.
To address communication gaps, Telegram-bot was tested to be integrated for real-time wildfire alerts from the community members, complemented by mobile application with online and offline capabilities of reporting.
Collaboration extended to the Government of Telangana included participation in a round table conference showcasing innovative technological solutions. A significant collaboration with the Centre for Wildfire Research at Swansea University played a crucial role in advancing the system’s enhancement.
This collaborative effort aims to contribute valuable insights to the wider community, aligning with the project’s commitment to knowledge-sharing and information exchange.
UNESCO invited DASH to the Ridge2Reef event, organised in collaboration with the Ministry of Environment, Forest and Climate Change of India, a platform that brought together environmental stakeholders globally. This event facilitated the exchange of experiences, challenges, ideas and best practices on a worldwide scale, involving participation from United Nations agencies.
DASH also garnered interest from the State Governments of Himachal Pradesh, Uttarakhand, Kerala and Chhattisgarh as well as other development partners. Facilitated by the APNIC Foundation, we also shared our project work with GSMA, who were keen to connect with organisations working in AI and Disaster Preparedness, particularly forest fires. DASH has also been selected for the innovative technology demo session at Global Digital Development Forum (GDDF), 2024.
Our commitment to knowledge exchange is evident in our regular interactions with Firefly, a fellow recipient of the Ian Peter’s grant, for continually exchanging learning experiences and exploring opportunities for collaboration.
Impact story
The implementation of the DASH unified system in the Nallamala Forest area of Telangana has seen changes in knowledge, awareness, attitude and behaviour among stakeholders.
Through the establishment of a technical working committee, stakeholders gained deeper insights into the applicability of AI/ML algorithms and IoT technology, thus enhancing their adaptability to the digital age. This has spurred engagement in technology-driven initiatives in forest management.
Through training sessions on the DASH app, beat officers gained insights into the use of the application and developed awareness about the role of technology in wildfire prediction, detection and mitigation. This has led to awareness creation on technology as a valuable tool for protecting the environment.
Awareness programs have reinforced the dangers of wildfires among community members. The community has been made aware of the need to limit certain activities and work closely with beat officers to protect forest areas. While these social changes may take time to demonstrate measurable impact, they are crucial steps towards long-term sustainability.
The project has also helped in encouraging a wider conversation with critical stakeholder mainly with Ministry of Environment, Forest and Climate Change, State Department of Forest, India Meteorological Department, Research Institutes such as Indian Institute of Forest Management and development partners such as World Bank and UNDP in the possible use and scale for technology-enabled platform like DASH for prediction, detection and mitigation of wildfire and also similar disasters. With climate change, the need for early warning systems is critical.
Project Sustainability
As outlined in our sustainability plan, we have adopted a human-centered approach, ensuring that the solutions developed are collaboratively designed with the stakeholders. This approach aims to tailor the solutions to the specific needs and context of the stakeholders, fostering greater acceptability and long-term sustainability. The project team has established a close working relationship with forest officials, particularly the beat officers who are integral members of the local community.
With the vision to strategically use technology in wildfire management, it requires planned approach for full-scale operationalization of the system at the ground level. This requires working closely with the forest department to adequately plan for an overall organization change management exercise, strengthening their capacity to further leverage control center, technologies for daily reporting etc.
As part of the pilot, training sessions were organized with beat officers and additional forest officers and contractual community staffs. This collaborative and engaging activity not only facilitated seamless transfer of knowledge but also exemplified a significant stride towards inclusivity. Notably, the training sessions saw active participation from women, underlining our commitment to fostering diversity and ensuring that the benefits of the project are shared inclusively among all community members. This human-centered and inclusive approach lies at the core of our sustainability strategy, promoting community ownership and long-lasting positive impact.
The project has indeed generated opportunities for future development and growth. The scalable nature of the solution, applicable to regions with similar landscapes and climatic conditions, has garnered interest from various governments and development partners. Ongoing discussions and engagements are in progress to explore new funding opportunities, partnerships and sponsorships that can support the expansion of similar initiatives in different geographies.
Lastly, the project activities are an ongoing process, and several key components will persist beyond the grant timelines. This strategic continuation is essential to ensuring the continuous development of the solution and maintaining its sustainability. By fostering strong relationships with local stakeholders, including forest officials and beat officers, and actively seeking partnerships and funding opportunities, our organization is committed to upholding the positive impact of the project in the long-term. This approach aligns with our sustainability plan, emphasizing community ownership and the enduring success of our initiatives.
Project Management
The project administration, staffing, and procurement activities have demonstrated a proactive and adaptive approach, contributing significantly to the fulfilment of project objectives.
To ensure effective project administration beyond our standard procedures, we established dedicated project management teams tasked with overseeing various aspects of the project lifecycle. These teams have been instrumental in coordinating activities, tracking progress, and mitigating risks. We also identified the need for dedicated on-ground staff for continuous monitoring and providing support in local language. Community youth who have enlisted themselves as fire watchers were particularly keen to support the activity roll-out. Our team members were on ground during all stages of the ongoing pilot activities, to ensure ease of communication as well as the necessary coordination and approval for the activities.
We implemented streamlined communication channels and regular reporting mechanisms to facilitate transparency and accountability across stakeholders. These efforts have enhanced efficiency and facilitated timely decision-making, contributing to the overall success of the project.
Throughout the project’s course, the numerous visits and conversations with the forest department have undeniably sparked transformative changes within our organization. Conversations and site visits revealed overlooked factors in forest preservation, prompting a shift in our own behaviours. Recognizing that small actions can have significant impact, we have embraced a heightened consciousness towards environmental preservation, wildlife conservation and community welfare. This awareness fuels our dedication to responsible and sustainable practices.
The pilot project has indeed inspired change within our organization by fostering a culture of innovation, collaboration, and continuous improvement. Through cross-functional teams and interdisciplinary collaborations, we have leveraged diverse expertise and perspectives to tackle complex challenges and drive meaningful impact. Moreover, the adoption of cutting-edge technologies and best practices has stimulated creativity and sparked new ideas for future initiatives especially extending the DASH unified platform for use cases for unique forest challenges.
The project has played a pivotal role in consolidating our organization’s reputation and strengthening its credibility within the broader community. By demonstrating our commitment to excellence, innovation, and sustainability, we have earned the trust and confidence of key stakeholders, including government agencies, industry partners, and civil society organizations. Furthermore, the successful ongoing implementation of the project has showcased our capacity to deliver impactful solutions and address complex challenges effectively.
Project Recommendations and Use of Findings
The findings and lessons learned throughout the pilot have played a pivotal role in shaping our decision-making and development processes.
During our research, it was identified that 95% of the fires are man-made, accidental or due to community practices. Consequently, a crucial behavioural research component was introduced and is ongoing to comprehensively understand human actions and correlate them with fire instances.
Some of the recommendations that can be shared based on the lessons learned so far are:
● Understanding the vision and need of the organization (in this case, the State Department of Forest). The aim is to support them with a system that complements their work and leads to positive impact.
● Emphasizing proactive community engagement strategies for local and visiting tourists, to encourage diverse participation.
● Understanding regulatory processes and specifics do’s and don’ts. The forest is a protected area and compliance is mandatory.
● Fostering a culture of efficient information-sharing among government departments to mitigate delays.
With interaction with other stakeholders, that the platform may be leveraged across different use cases for effective utilization of resource. For example, the reporting application may be used for sending reports not only on wildfire but other wildlife risks (poaching evidences, human-animal conflict, illegal logging etc.) and also use comprehensive dashboard based on indicators and roles.
As we work complete this amazing pilot journey, we take forward these learning to embark on future planning. The experiences gained shall contribute to the development of more resilient and adaptable approaches in the evolving landscape for wildfire prevention and management and also management of other forest crisis for holistic early warnings.
Bibliography
2. https://www.un.org/en/climatechange/early-warnings-for-all
3. https://www.sciencedirect.com/science/article/pii/S0277539515301783
4. https://www.oecd.org/dac/gender-development/44750309.pdf
This work is licensed under a Creative Commons Attribution-ShareAlike 4.0 International License